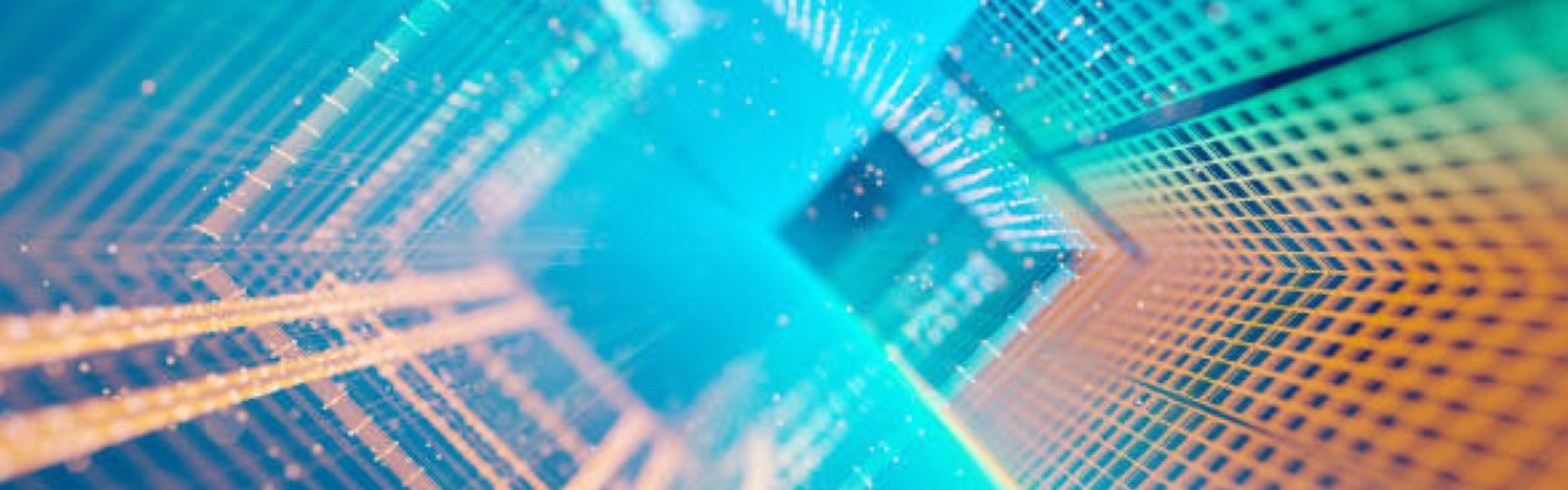
How Will Automation Change Enterprise Data Science? – Part 1
Thought Leadership
According to a recent study by Dimensional Research, Over 96% of enterprise companies struggle with AI and Machine Learning (ML) projects. The reasons behind the incredibly high failure rates are numerous, but many are associated with shortages of staff, data that requires too much pre-processing to use appropriately, and a lack of understanding of the ML models on the part of business users. Organizations struggle with AI and machine learning, in large part, because projects take too long to complete, lab-generated results are often difficult to recreate in production environments, and the value derived by AI and ML projects is not clear enough.
AI & Machine Learning Automation – The Solution To All Our Problems?
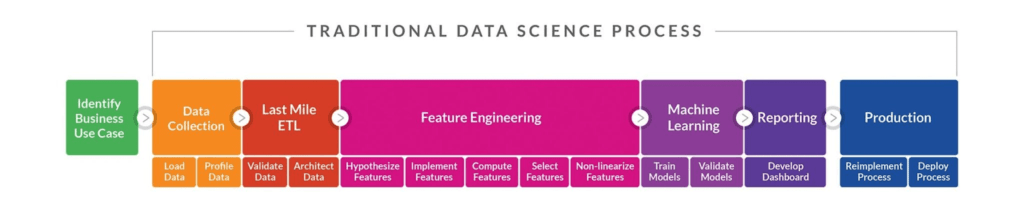
Recently, several startups have come to market with innovative platforms designed to “automate” the process of generating machine learning models. The promise of “AutoML” as it has become known, is that by accelerating the machine-learning part of the process, work accelerates, quality increases and (hopefully) eliminates many of the challenges associated with AI and machine learning. AutoML is tackling one of the critical challenges that organizations struggle with: the sheer length of the AI and ML project which usually takes months to complete (and many of them fail!), and the incredible lack of qualified talent available to handle it. In fact, according to a 2018 study published by LinkedIn, there is a national shortage of over 150,000 data science-related jobs. Compounding the problem, the very nature of data science is iterative, time-consuming, and confusing.
While current AutoML products have undoubtedly made significant inroads in accelerating the AI and machine learning process, they fail to address the most significant step, the process to prepare the input of machine learning which we refer to as “last-mile” ETL and feature engineering. Enterprise data are stored for business purposes in many tabular tables with complex relationships and are not ready for machine learning, which requires a single flat aggregated table. The process to transform the raw business data into the flat “feature” table requires wrangling, cleansing, joining, combining, aggregating data to identify data relationships and extract relevant patterns to build ML models. This data and feature engineering are a heavily iterative and time-consuming process requiring multiple resources.
Raw business data are often lower quality, more substantial, more complex than ML-ready data. More importantly, domain knowledge is the key to identify relevant patterns to make ML models work. It is essential to know that what makes ML models work is neither how to tune ML models nor which algorithms to use. It is what to input to ML models (a.k.a. garbage in, garbage out). In other words, the quality of the last-mile ETL and feature engineering process govern the performance of ML models. The single most significant gap in all currently available AutoML products is the lack of automation of last-mile ETL and feature engineering processes that require heavy involvement from data scientists, data engineers, and domain experts and that are very time-consuming.
Data Science Automation, A New Way For AutoML
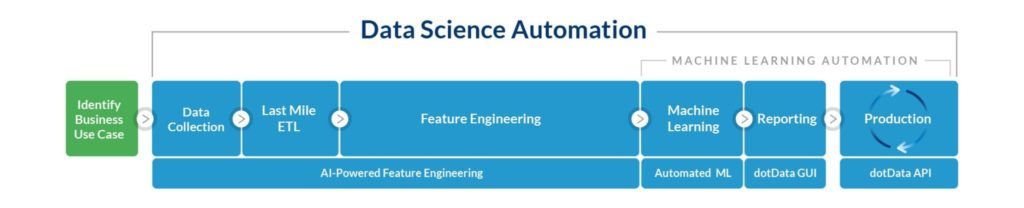
At dotData, we firmly believe that to create a genuine shift in how modern organizations leverage AI and machine learning, the full cycle of data science development must involve automation. If the problems at the heart of data science automation are due to lack of data scientists, poor understanding of ML from business users, and difficulties in migrating to production environments, then these are the challenges that AutoML must also resolve. It all begins with automating the last-mile ETL as well as the Feature Engineering that are the most time-consuming and most iterative parts of the AI and ML process.
Any AutoML tool must provide automation capabilities that go beyond just “vetting” features, but that can also create features automatically and presenting the information in an easy to consume, easy to digest manner that non-experts can leverage and understand. Of course, once features are automatically generated and presented, your AutoML solutions should also provide ways of testing and ranking multiple machine learning algorithms, whether built in-house or whether they leverage popular ML techniques like XGBoost.
To provide for a fully automated solution; however, the migration of AI and ML models into production is also a critical last step that must provide automation. One of the biggest challenges of implementing AI and ML models is that what worked perfectly in the data science lab often has problems in real-world environments. Failures of AI and ML models in real-world environments create a time-consuming loop involving data scientists and IT specialists who must identify problems that have arisen during implementation and attempt and deploy fixes.
By implementing AI and ML models using an API-based approach, companies can “push” AI and machine learning models into productions while still maintaining a direct connection to the automation platform that generated those models. By leveraging an API connection of the automation platform, the features and ML models can be maintained along with data changes and degradation of the predictive performances.
Stay tuned for Part 2, published next week…