Manage Data, Features, and ML Predictions in A Single Pipeline
dotData Ops empowers BI and analytics professionals by providing an intuitive, self-service environment for efficiently deploying and operationalizing data, feature, and prediction pipelines. Through dotData’s automated feature discovery technology, revolutionizes MLOps – monitoring the Business Impact of your features and models, diagnosing source data for enhanced insight into feature drift, and re-engineering features to combat data drift beyond model refitting.
Rapid Business Validation With Self-Service Feature & Model Deployments
Moving from Proof of Concept (PoC) to operationalizing models involves challenges in system integration, data migration, and changes to business workflows. dotData Ops addresses these challenges by enabling simple, rapid deployment of data and feature pipelines in addition to ML models. dotData Ops offers an agile, self-service platform for analytics teams to swiftly deploy and validate pipelines in real-world scenarios for quicker investment decisions and boosting business leaders’ confidence.
Step 1
Deploy models in seconds
Upload your ML model package for rapid, easy deployments.
- Instantly deploy streamlined pipelines of data, features, and ML predictions with a simple package-based deployment.
- Specify your data locations to start feature generations automatically and predict and monitor performance.
Step 2
Schedule prediction jobs with a few clicks
Configure regular predictions using deployed models through an intuitive GUI.
- Schedule regular prediction jobs with a few clicks for daily, weekly, monthly, or fully customized schedules.
- Maintain optimal infrastructure costs with cluster auto-scaling in scheduled jobs.
- Receive status notifications model & feature quality alerts on Slack or Microsoft Teams.
Step 3
Monitor the business impact of models & features
Monitor and visualize the business impact along with quality and performance of your models and features.
- Track business, model, and feature quality metrics on a single dashboard.
- Visualize business and technical performance metrics to ensure your models continue to deliver value over time.
- Detect and receive alerts of degradation of models and features to take immediate preventive actions.
Step 4
Trace the root cause of model & feature drift
Diagnose the root cause of model or feature degradation by tracing problems back to source data.
- Discover the key features responsible for the decline in model performance and analyze how their distribution and statistical metrics have changed over time.
- Trace the root cause of model and feature drift back to source data and diagnose whether errors or essential data changes are responsible.
Step 5
Feature re-engineering to combat drift
Move beyond simple model retraining by re-engineering features to combat data drift.
- Re-engineer features to maintain model and feature quality in response to essential changes in your source data.
- Stay ahead of data changes by continuously discovering and delivering new features and insights.
Product Features
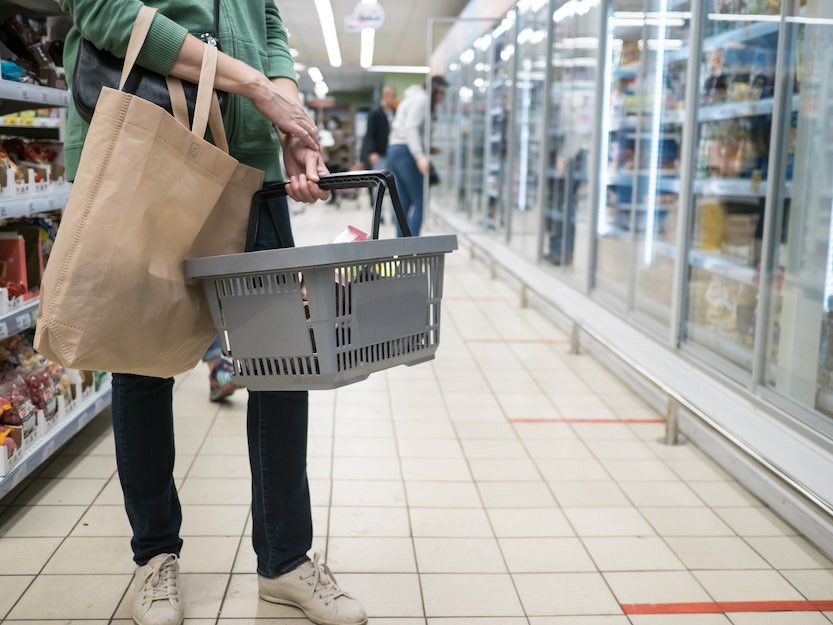
How Retail Chain Lawson Increased Sales 12X with Feature Discovery
Download our latest case study to see how global retail chain Lawson leveraged the power of predictive analytics and dotData’s unique Feature Discovery technology to build a predictive analytics-driven marketing campaign that resulted in 1,200% sales growth.
See it in action
Book a quick consultation to see if dotData Ops can help you take your MLOps plans to the next level.
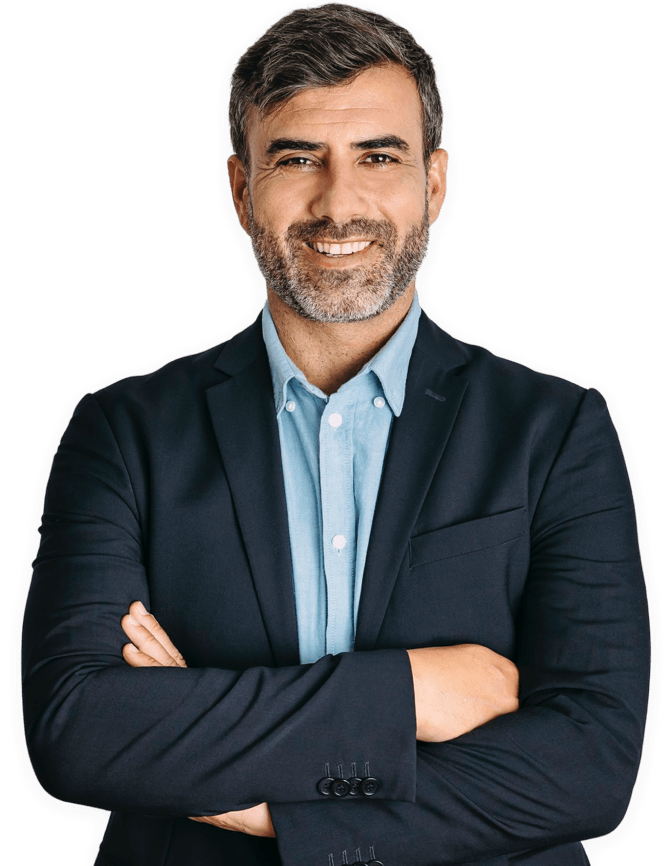