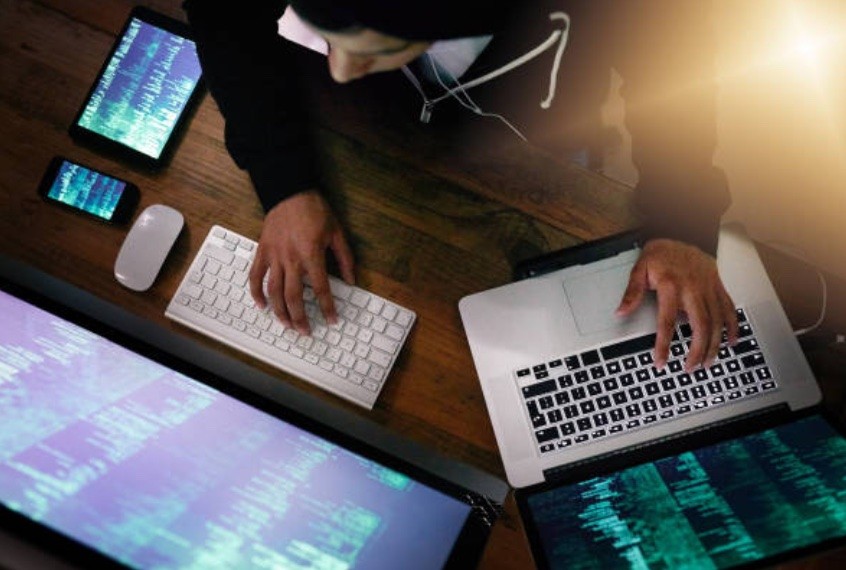
Take Advanced Analytics into Overdrive with AutoML 2.0
The term “Advanced Analytics” was coined by the Gartner Group and is defined as the “…autonomous or semi-autonomous examination of data or content using sophisticated techniques and tools, typically beyond those of traditional business intelligence (BI), to discover deeper insights, make predictions, or generate recommendations.” Advanced analytics, by definition, requires the use of advanced techniques like data mining, machine learning, pattern matching, and other sophisticated manipulation of data in an effort to gain greater insights. The most broadly used category of advanced analytics is also known as predictive analytics. Predictive analytics itself is not new, but has traditionally been the exclusive domain of data scientists and highly skilled statisticians due to the extremely complex mathematical models required to effectively build predictive dashboards. While many organizations can benefit from predictive analytics, only a few are able to create and deploy dashboards powered by predictive algorithms, due to the high cost of hiring and retaining talent.
Why Advanced (Predictive) Analytics?
The benefits of advanced analytics and predictive analytics are relatively intuitive, given the typical use cases where predictive modeling can be beneficial. Customer churn, for example, is one of the most highly used and beneficial use-cases for predictive analytics. Predicting which customers are likely to churn can provide a business with an increased focus to be able to target those customers for upgrades and promotional offers, lowering churn rates. Similarly, predicting the likelihood of default on loans or outstanding payables can provide huge savings to organizations by limiting exposure to long-term collections. In marketing, predicting the likelihood of a campaign’s performance can have a massive impact on return on investment and can help marketing teams provide better focus for their efforts. Even as early as 2011, research firm The Aberdeen Group found that businesses using predictive analytics could identify the right target audience and make precise offerings to them at twice the rate of companies that were not using predictive analytics. The benefits of being able to predict business outcomes is tangible and of high value. The challenge, historically, has been that developing predictive analytics systems has been difficult, time-consuming and expensive.
The Traditional Workflow of Building Predictive Dashboards
When most people think of predictive analytics, the first thought that comes to mind is “expensive.” For most organizations, the challenge of predictive analytics is the cost involved in building out effective models that are delivered in business-friendly dashboards that can be used by line-of-business users. The reason achieving a well-formed predictive workflow is challenging is because of the steps involved in going from “data” to “predictive models.” Fundamentally, there are 5 steps involved in moving from just having “data” to using it to predict business outcomes:
- Data Collection & Consolidation – Anyone who works in a large enterprise organization knows that enterprises love data. The problem, however, is that data lives in silos – separate systems for sales, marketing, operations, accounting etc. – sometimes sharing data – sometimes not. The first challenge of moving from data to predictions is that you need to take all that data and consolidate it into a unified analytics platform that can provide all relevant data for you to use and analyze.
- Data Prep and Data Cleansing – Another major challenge in preparing data for predictive analytics is often referred to as normalization. Data normalization has two distinct phases – first, data must be unified and normalized across systems – this is typically a highly manual process that involves performing actions like ensuring that field values are consistent across systems and that duplicate data is removed from the final data warehouse. The second phase is preparing data for AI modeling – also often manual and designed to minimize errors in AI model development due to problems with the data.
- Business Hypothesis Ideating, Testing & Validation – Once data is ready for analysis, the predictive analytics process requires what are commonly referred to as “features.” Features are nothing more than ways of using data to describe a potential useful outcome. Features will, in turn, be used in machine learning and AI models to derive predictions. Feature generation is often very time-consuming and manual and requires input from subject matter experts as well as data scientists and engineers in order to create, test and evaluate the usefulness of individual features.
- ML Model Development and Testing – With features built, the next step in the process is to use features to test against multiple ML algorithms to test which ML models might provide better results. Again, this can be a very iterative and time-consuming process. In recent years, software tools known as “AutoML” have made the process of evaluating and testing ML models more automated.
- Deploying Models into Production Environments – Once a model is built and has been tested, the next phase is to deploy the model into the ultimate production environment. For BI-based predictive applications, this might be a PowerBI dashboard or a Tableau dashboard that provides some form of predictive scoring based on user input (filters, drop-downs, etc.), allowing users to perform “what if” analysis on the business problems being predicted.
AutoML 2.0: Automating Your Workflow
The five steps outlined above are actually a fairly simplified version of what actually tends to happen. There are multiple steps where manual work involved requires multiple experts and multiple types of work. While AutoML 1.0 tools have allowed for the rapid development of Machine Learning models, they have still relied on prepared data. New platforms, however, are becoming available that can automate nearly the entire process – from AI-based data prep all the way to model deployment, allowing for the first time BI teams to develop, test and deploy predictive models without having to hire expensive data scientists. These AutoML 2.0 platforms are ideally suited for mid-sized organizations and smaller enterprises that can benefit from predictive analytics, but may not have the data science skills or staff to execute on traditional workflows.
Next Steps
So how do you get started? As a first step, it’s important to understand the core differences between AutoML and AutoML 2.0 platforms. Investing in the wrong type of product could create a nightmare scenario where additional staff and new software packages are required before you can create value from your advanced analytics infrastructure. Organizations serious about advanced analytics must leverage data science automation to gain greater agility and faster, more accurate decision-making. The emergence of AutoML platforms allows enterprises to be more nimble by allowing them to tap into current teams and resources without having to recruit and additional talent. AutoML 2.0 platforms empower BI developers and business analytics professionals to leverage AI/ML and add predictive analytics to their BI stack quickly and easily. AutoML 2.0 platforms not only generate features automatically, eliminating the most complex and time-consuming part of workflow, but also select the best algorithm depending upon the application. By providing automated data preprocessing, model generation, and deployment with a transparent workflow, AutoML 2.0 is bringing AI to masses thereby accelerating data science adoption.