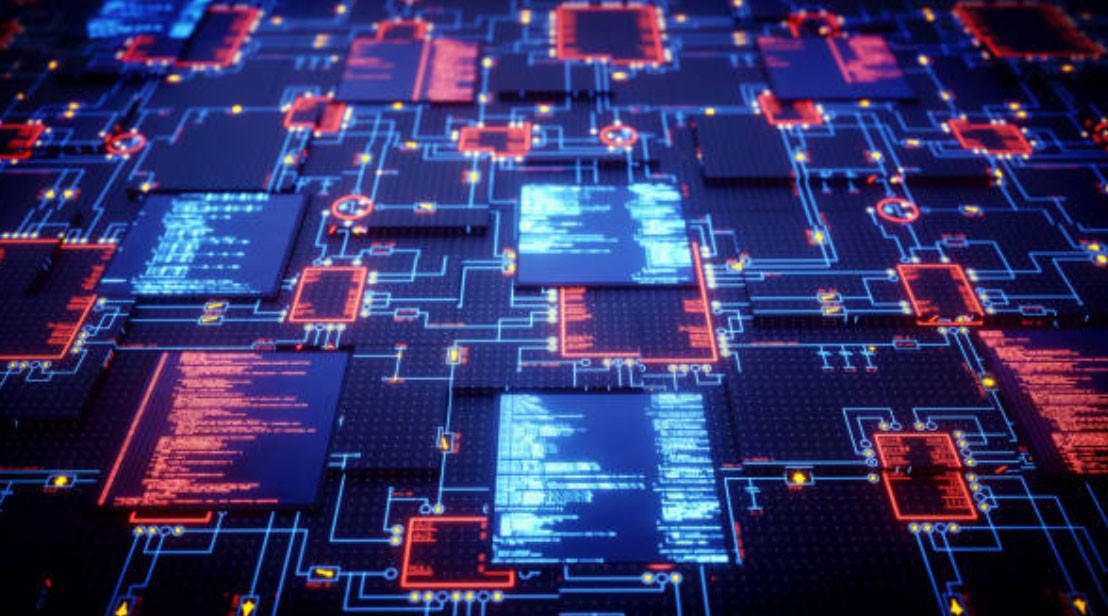
What IS Feature Engineering?
- Thought Leadership
The past few years have seen the rapid rise in the adoption of Artificial Intelligence (AI) and Machine Learning (ML) for a multitude of commercial use-cases. Beyond the “cute” factor of AI that can pick a cat out of a photo array, AI and Machine learning are being deployed to model and predict lending risk, to understand and manage customer churn, provide product recommendations, help with programmatic advertising and much more. The challenge for the business community is that the underlying practice that is at the heart of AI and Machine Learning – data science – is rooted in a complex world of statistical analysis, data manipulation, programming and more. Most businesses don’t have enough data scientists – a fact illustrated by research in 2018 by LinkedIn that showed that there would be a shortfall of over 150,000 people with data science skills in the US alone. The data science process is complex and involves multiple distinct phases, as illustrated below. A typical data science project can take months to complete – with the most complex part being the feature engineering piece.
Surprisingly, even in our daily conversations with clients, we find that there is often some amount of confusion as to what the term “feature engineering” actually means. What exactly is feature engineering? What are the steps of the process and why does it take so long? What can we do to accelerate this process? At a most basic level, feature engineering is comprised of three distinct steps:
The first two steps in the process, feature ideation and feature selection, often require a high degree of “domain knowledge.” Domain knowledge refers to knowledge of the underlying business requirements that must be addressed. For example, a bank might employ a team of business analysts and data analysts to work with the data science team to consider “features” that might be useful in predicting if a client is likely to convert on a “zero balance” transfer offer for a new credit card. During this phase, a high degree of analysis of data is required to understand what data sources, tables and columns might be used to create the “features” that will then be tested in the next phase.
Feature creation and testing are the next part of the process. During this phase, data scientists collaborate with business analysts and data engineers to create flat tables that combine data from multiple related tables in one single “feature table.” For example, the same bank in our previous example might take data from their web tracking system, from their customer records, and from other data sources to create a single table that provides data for individual prospective clients that might be used by a machine learning model to predict the likelihood of that consumer accepting an offer. Each feature that is created must then be evaluated against machine learning models to identify which feature/model combinations provide the best possible outcome.
Clearly, the process of feature engineering can be lengthy, time-consuming and resource-intensive. Most organizations simply don’t have enough talent or time to effectively evaluate all possible use cases and to evaluate all possible permutations and combinations of tables and columns of data. Automated Feature Engineering can provide a huge benefit to businesses that aim to leverage AI and ML models for their business. The word “automated feature engineering,” however, can often mean different things, depending on which vendor you are evaluating. For most providers of Automated Machine Learning (AutoML) software, “automated feature engineering” describes the process of evaluating which features – built manually using the process described above, will be most beneficial for any given machine learning model. True Automated Feature Engineering, however, leverages Artificial Intelligence (AI) to create and evaluate features automatically. This is why at dotData we talk about discovering the “unknown unknowns” using Automated Feature Engineering. By automating the entire feature building process, you can build and evaluate hundreds of thousands, potentially even millions of features automatically – exposing only the ones that pass a specific threshold – and then providing data scientists with a wealth of additional features that they may have never considered.
To be specific, Automated Feature Engineering is not a replacement for manual feature creation and evaluation but instead can provide two significant benefits: Rapid prototyping and feature augmentation. Automated Feature Engineering can be used by data scientists to accelerate the process of trial and error that is often associated with feature engineering. Feature augmentation, on the other hand, is the process of using Automated Feature Engineering to create additional features that the data scientists, business analysts and data engineerings might have never even considered.
What are the benefits of Automated Feature Engineering? By far the most valuable benefit is that of accelerated performance. Many dotData clients have leveraged the Automated Feature Engineering features of our dotData Enterprise or dotData Py platforms to accelerate their data science processes, often being able to deliver in days what traditionally took five months or longer to deliver. With the exponential growth in need for AI and ML use-cases and the low availability of data science resources, Automated Feature Engineering – as part of an effective AutoML platform – can help businesses grow exponentially the number of AI and ML projects that are executed and successfully brought into production.
Learn more about our platform and about Automated Feature Engineering by visiting our website.