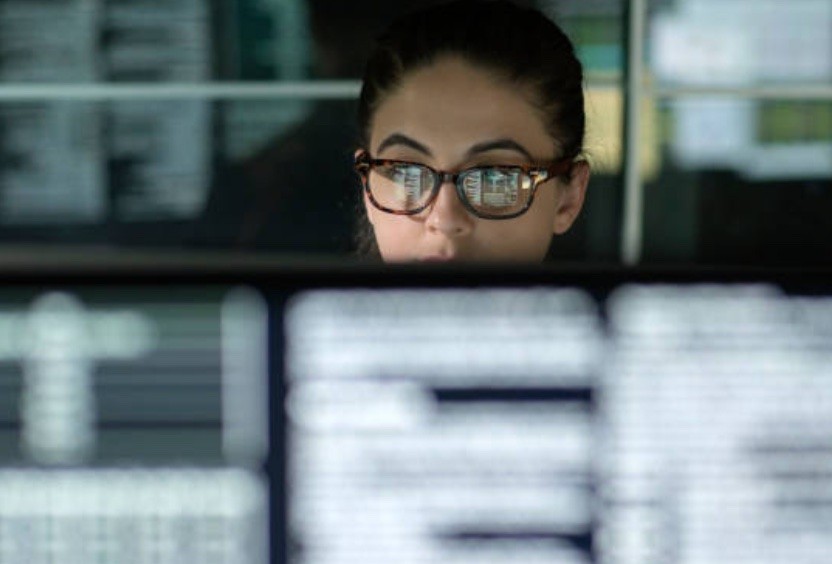
Why Your Company Needs White-Box Models in Enterprise Data Science
Our CEO Ryohei Fujimaki, Ph.D., explains the benefits of white-box models in Enterprise Data Science @AIWorldExpo #AI Trends:
AI and Machine Learning are accelerating digital transformation initiatives across multiple industries. When fully operationalized, AI and ML enable organizations to make data-driven decisions at unprecedented speed, transparency, and accountability levels. White Box Models (WBMs) render prediction results and influencing variables easily interpretable, such as linear models and decision/regression tree models. Data scientists create complex features using complex mathematical transformations and deep learning algorithms, but such models are difficult to explain from customer behaviors. Modeling for business problems has implications for three key personas: model developers, model consumers, and business units or organizations.
Transparency levels depend on the nature of the business. The lowest level is the black-box model, which cannot provide insight into the model’s workings. Linear and decision tree models are White Box models. Black-Box models are harder to understand and are hard to explain using approximation techniques. Interpretability of ML models is essential but not enough to be accepted by business users. AutoML is gaining momentum and empowering a broad base of professionals to create AI/ML. To implement AutoML 2.0 model and feature transparency must be given in understandable representations for human experts. Modern data science applications require white-box models. White-box models allow organizations to stay accountable for their data-driven decisions. Read more at AITrends.