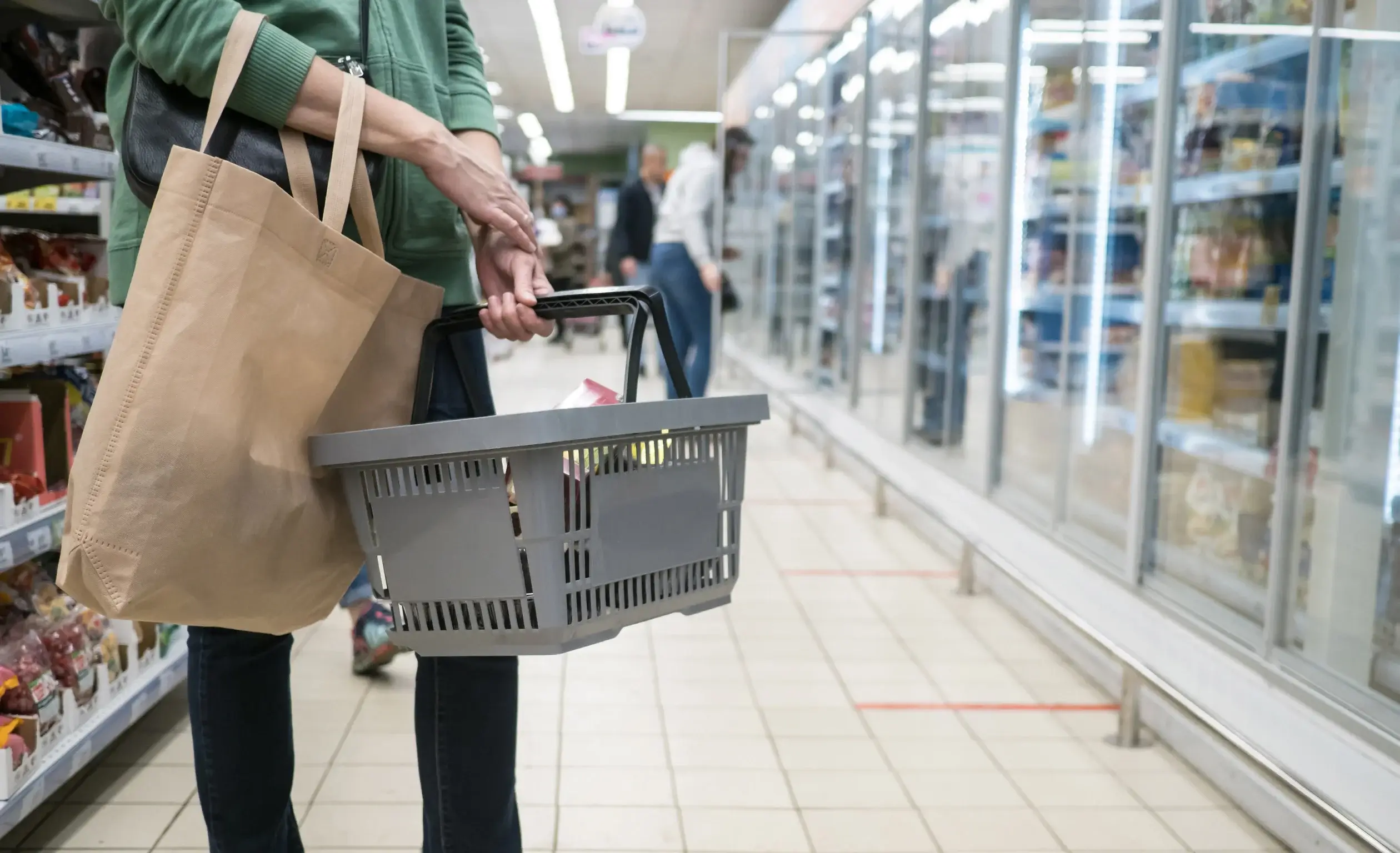
How Retail Chain Lawson Increased Sales 12X Through Feature Discovery
Global retail chain grows sales 1,200% with coupons targeted around core client values & segments.
Industry: Retail
Solution: Ad Design Optimization
Lawson, a global retail chain that operates 15,000+ stores, introduced dotData Feature Factory to power a marketing system to create targeted campaigns. In response to the new realities of the cookie-less era, Lawson wanted to leverage their wealth of Point of Sales (POS) data linked to client IDs. The Lawson team analyzed and used this “ID-POS” data to extract features based on customer value. By optimizing coupon design to match the features identified by dotData, Lawson was able to increase sales by 12X. The features extracted by dotData are used internally to improve store sales by increasing the lifetime value (LTV) of members who purchase goods from Lawson. The features derived from the ID-POS data are also used for marketing and product design and planning activities for manufacturers, extending the benefits of the program to Lawson partners.
Challenges
- Typical customer segments, such as gender, age, and past purchase history, were not enough to promote their products’ uniqueness.
- The vast amount of POS data made performing analytics and predictions difficult and it was becoming hard to influence customers.
- They needed measures to target new customers without relying on cookies.
Solutions
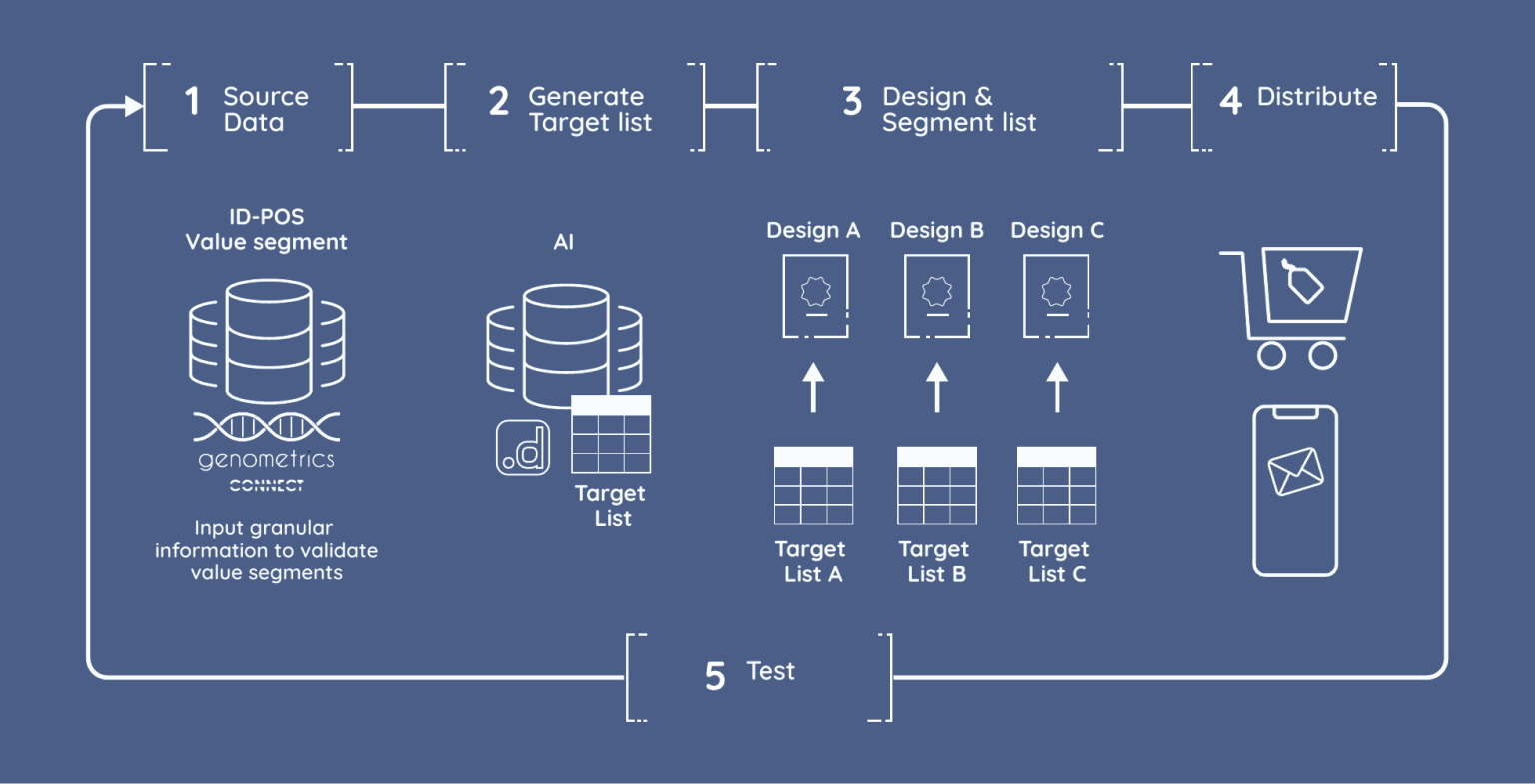
- dotData’s proprietary Feature Engineering technology evaluated billions of data points across multiple categories – simultaneously.
- The ability to discover features across multiple tables meant Lawson could use ID-POS data to build highly targeted features.
- dotData’s unique transparency provided the business with explainable insights that business users could understand.
Results
- Extracted features from ID-POS data and the customer’s value, and segmented customers according to their values.
- Increased sales by 12X by re-designing coupons based on dotData discovered features.
- They were able to monetize their new cookie-less data management model.
What Our Customers Say
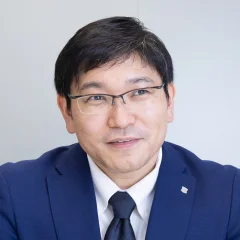
Toshiro Kobayashi
Data marts need to be updated continuously, for data changes, new data additions, new product trends, etc. The solution came from dotData, which automatically generates data marts and automates feature discovery and extraction with its own technology.
Business Background and Challenges
Lawson operates a chain of franchise convenience stores. The company continues to innovate and enhance its products and services by quickly identifying social issues and by adapting to rapidly changing customer values. As of the end of February 2022, the company has 14,656 stores in Japan.
Lawson operates convenience stores nationwide and responds flexibly and quickly to a wide variety of customer needs. They are trying to develop “value-based targeting” based on purchase history and information from their membership data.
“By understanding what customers value accurately, we can recommend products that match individual needs, improve our stores, and use customer data to support manufacturers’ product development and promotion activities,” says Mr. Kobayashi of Lawson.
This project started in 2015. “In retail, a product’s performance is judged primarily by unit sales,” says Mr. Kobayashi.
Even if a vendor took time to develop an outstanding new product, if it did not sell well, it would be considered a poorly performing product and be discontinued before consumers could become familiar with the product. This was a very frustrating issue for vendors and for Lawson.
“Signals-based targeting” uses a purchasing and sales model that extracts information about what customer find of value from a vast amount of historical purchase data to learn how consumer desires are reflected in the products they buy, leading to enhanced coupon design and distribution, targeted sales promotions, and precise product development.
Specifically, the company extracts the behavior and characteristics of customers from their vast point of sale data and product information and uses cluster analysis to extract and categorize consumer buying signals.
It is important to divide them according to their desires because what they want varies depending on multiple factors even when age and gender are unchanged.
For example, signals associated with what Lawson calls “women who reward themselves” describes young women who like to treat themselves and practice self-care.”
Signals are made up of multiple factors. So far, the challenge has been to scrutinize the items required for analysis from various data sources, such as ID-POS, the product master group, and the membership master group including signal classification, and to organize signals into data marts.
The maintenance of the data mart was manual and this artisanal approach took many man-hours and did not allow enough time to analyze the relationship between consumer signals and purchase patterns.
“Data marts need to be updated continuously, for data changes, new data additions, new product trends, etc. While we need to do targeted marketing on more products, outsourcing it to an external data scientist was challenging in terms of flexibility and cost” says Mr. Kobayashi. The solution came from dotData, which automatically generates data marts and automates feature discovery and extraction with its own technology.
Dotdata as Accurate as a Data Scientist
Automation was essential due to the lack of internal resources and because outsourcing would limit Lawson’s flexibility to modify data marts. Automation, however, would be meaningless if the quality of features extracted by dotData was low and imprecise.
Before the implementation, the team compared dotData against a large external data analysis consulting firm by providing an equivalent challenge to compare the analysis results. dotData was able to yield analysis results as accurately as top Notch data scientists – but with significantly reduced manpower.
The driving factor for Lawson’s decision to select dotData was the platform’s flexibility when making changes and the ease and flexibility for adding new data sets. Lawson is accumulating data daily from approximately 15,000 stores nationwide. Extracting the feature volumes needed to analyze this in detail and creating data marts were major challenges, and dotData was the best solution.
The company has integrated dotData into a custom developed user-friendly dashboard, giving department personell access to the power of dotData insights without needing to increase human resources and technical staff. The ease of use made it possible for marketing people with no knowledge or experience in data analysis to create predictive models by simply entering a few required pieces of information and by selecting parameters. By adapting the system for use by business users, the Lawson team minimized the risk of low usage because of lack of deep analytical skills or lack of technical resources. Ease of use is critical for advancing data utilization. If casual users who need to perform data analysis can access the results, the data becomes even more valuable and will be used broadly.
A “Data-Driven” Coupon Design
“Desires-based targeting” using dotData was incorporated into Lawson’s BI (Business Intelligence) tools for sales promotion, product development, and collaboration with partner manufacturers.
In 2021 they collaborated with a major confectioner to optimize a targeted marketing campaign.
A designer created multiple coupon designs that were based on the relationship between what the customer desired and what they purchased. These coupons were distributed to approximately 200,000 customers and each customer’s coupon design was based on the predicted scores generated by dotData. As a result, the purchase rate increased by a factor of 400% due to improved targeting accuracy based on predictive scores and by a factor of 300% due to design optimization based on consumer desires a 1200% total – demonstrating the huge impact desire-based targeting using dotData.
“We started creating this signal-based targeting marketing strategy based on the hypothesis that if we can target customers based on what they value, we will be able to promote the product based on what they will find appealing and increase sales. And this case proved that value-based marketing is more effective than marketing based on age and gender” says Mr. Kobayashi.
Preparing for the Cookieless Era
Going forward, Lawson plans to introduce a variety of signals-based targeting strategies. They are also looking into optimized targeting by linking membership data with smartphone applications, designing customer journeys, store operations, and opening new stores. “Instead of cookies, which are increasingly becoming less precise and more difficult to retreive, we believe that purchases in retail stores will be an important data point to help us understand customer desires,” says Kobayashi.
Furthermore, with the aforementioned example, Mr.Kobayashi says, “We are considering using it as a new data management model for the cookieless era. Since manufacturers cannot directly approach customers who are likely repeat buyers, we should be able to provide value by having data on the customer’s purchase behavior”. Using our data, we can create new revenue streams by promoting manufacturers’ product development and promotional activities. Finally, Mr. Kobayashi said, “The more data companies have, the more dotData can help them get the most value out of their data. As data analysis becomes more competitive for companies, we recommend dotData as the best solution for those who want to make their analysis in-house rather than outsource it to maximize the speed and have the analysis flexibility.”
Lawson
https://www.lawson.jp/en/Employees | 10,362 |
---|---|
Established | April 15, 1975 |