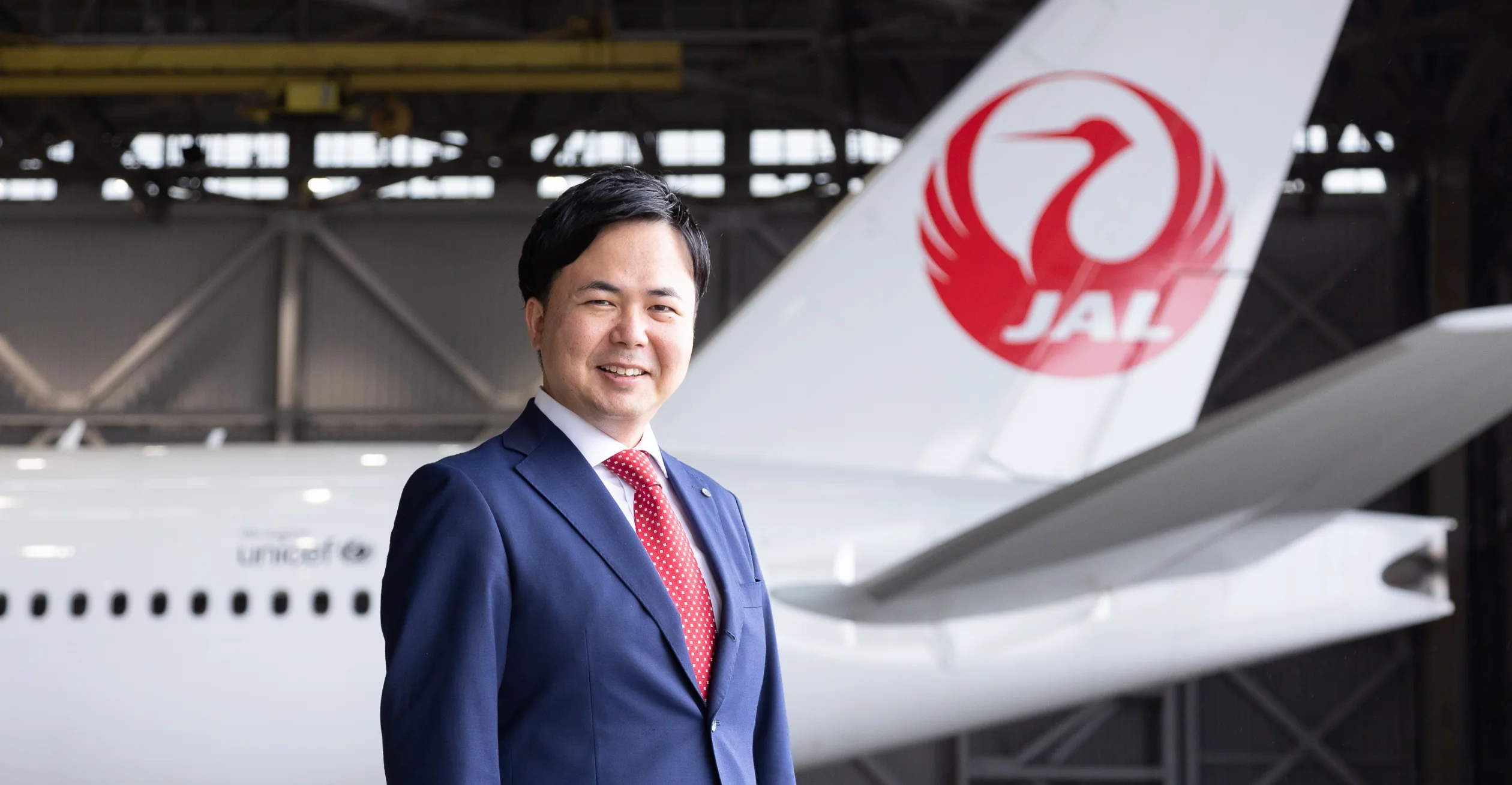
Japan Airlines Uses Predictive Analytics to Strive for Zero Delays
dotData features improve failure prediction analysis.
Industry: Airlines and Aviation
Solution: Predictive Analytics
JAL Engineering performs the maintenance of aircraft owned by the JAL Group. To minimize flight delays and cancellations due to aircraft malfunctions, the company is working on aircraft failure prediction by using big data analytics and introduced dotData in 2019 to strengthen its efforts.
In addition to predictions of failures that were previously detected based on the mechanic’s experience, more predictions can now be made by analyzing in-flight data and maintenance data. By strengthening these initiatives, JAL aims to achieve zero delays and cancellations caused by aircraft malfunctions.
Challenges
• Hypothesis-testing analysis based on the experience and knowledge of mechanics and engineers has limitations in the ability to detect signs of failure.
• Many AI products are not suitable for failure prediction analysis since their results are derived through black-box models.
• Due to the vast amount of flight data, it took a lot of work to comprehensively verify patterns (features) that may be signs of failure.
Solutions
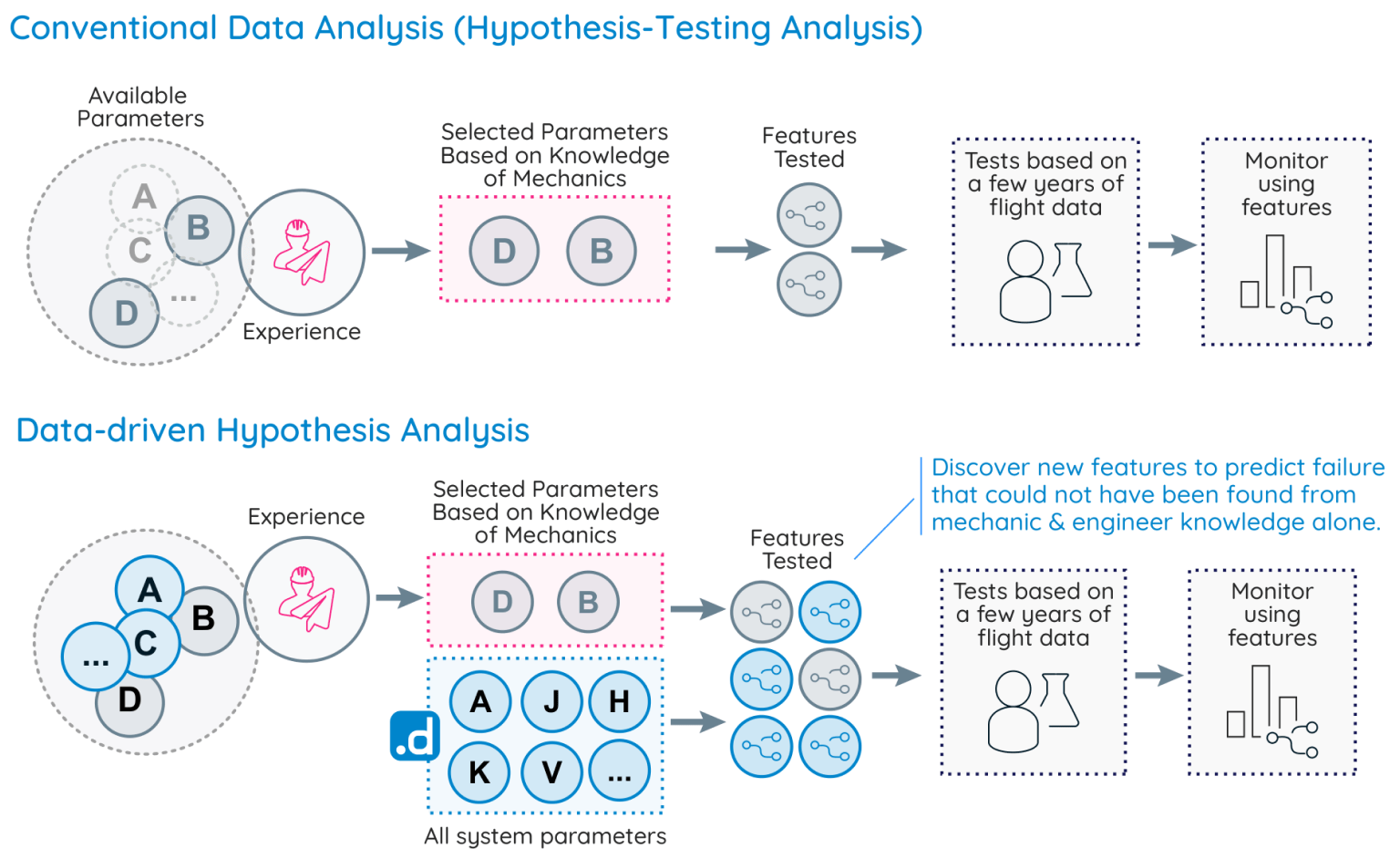
Results
- Enhance the overall failure prediction analysis by combining dotData with conventional hypothesis testing analysis.
- Successfully discovered features for detecting signs of failure that could not be found by hypothesis testing analysis.
What Our Customers Say
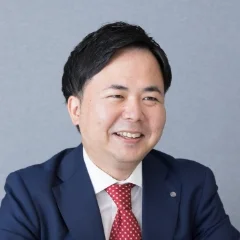
Toru Taniuchi
dotData allowed us to discover new features that lead to predictive failures that could not be found by hypothesis testing analysis based on the knowledge of mechanics and engineers.
Using Big Data Analytics to Predict Aircraft Malfunctions
JAL Engineering Co., Ltd. is an aircraft maintenance company that carries out maintenance of JAL Group aircraft and supports safe operation. In addition to JAL Group aircraft, the company also provides maintenance services for other airlines’ aircraft flying to Japanese airports.
The company is promoting various initiatives under the slogan “Zero Zero 100,” aiming for “zero irregular operations,” “zero inflight defects,” and a “100% on-time departure rate”. One of the main initiatives is the “Failure Prediction Project”. For many years, the company has been continuously improving the quality and efficiency of its maintenance operations to enhance the quality of its aircraft. One of the company’s most important efforts is “preventive maintenance,” which aims to identify and prevent defects before they occur.
In the past, preventive maintenance relied mainly on “five senses,” with mechanics checking the aircraft’s condition with their eyes and listening for unusual noises. In recent years, the company has been focusing on measures to analyze big data from the large amount of “flight data” acquired from sensors attached to aircraft, and to detect signs of failures. According to Toru Taniuchi, an analyst in the company’s Engineering Department, this initiative, called the “Failure Prediction Project,” began in 2016 and has so far produced several successful results.
“We analyze historical sensor data acquired from each flight and historical maintenance data together. Our method is to first formulate a “hypothesis” of a scenario leading to a malfunction based on the experience and knowledge of mechanics and engineers, then verify the hypothesis with the data for the period where the actual failure occurred and find “ specific features” in the data that indicate signs of the failure. We then verify whether we can detect the predictive signs by using these specific features with more broad historical data. This series of methods is called within the company as “hypothesis-testing analysis.” To date, this method has steadily produced results, including the creation of about 100 prediction models that can detect signs of failures.” says Mr.Tanicuhi.
Adopted dotData as the Tool for Extracting “Features”
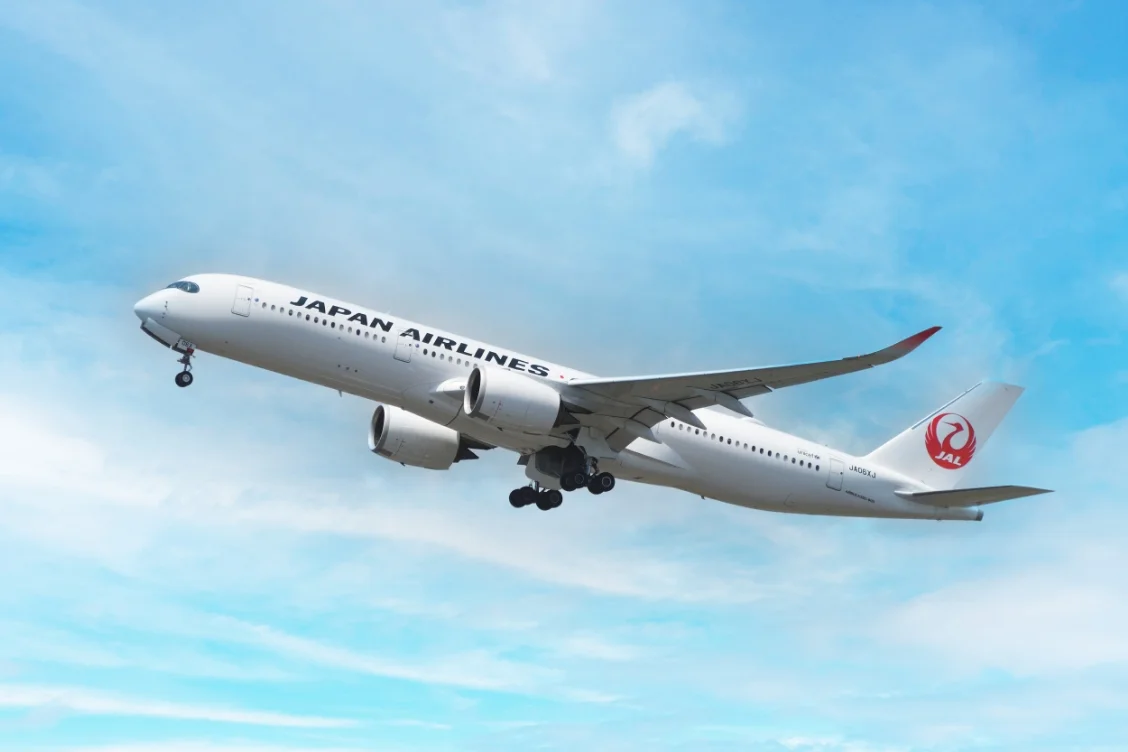
Relying on traditional means of discovering significant features was not successful in some cases. Especially for electrical system defects, it is difficult to establish failure prediction models using hypothesis testing analysis.
To solve this problem, the company developed a new approach called “data-driven hypothesis analysis”, which derives possible hypotheses from a given set of data. By using this new approach, there is a possibility that some signs of failures can be detected based on trends in sensor data and maintenance data, even for failures the hypothesis testing analysis couldn’t find the sign.
However, to perform a data-driven hypothesis analysis, it is necessary to find features in a vast amount of data, which is difficult to do with conventional data analysis tools. JAL then turned to dotData, which has a unique technology called “Automated Feature Discovery”.
“One of the data scientists who belonged to a different division of our company introduced our project team saying, dotData has the unique technology and you should give it a try. At the time, there was no other technology that could automatically find features in data and provide them in a form that could be interpreted by humans, so I thought, ‘This could be used for data-driven hypothesis analysis.” says Mr. Taniuchi.
The key to data-driven hypothesis analysis is the challenge of finding significant features in the data. At the time, other AI and machine learning tools and products existed, but in most of them, the feature values had to be designed and provided by humans, and the results tended to be black boxes. In contrast, dotData automatically extracts features and visualizes them on in software, so it exactly met the needs of the “data-driven hypothesis” failure prediction.
Developed an Original Method for Extracting Features
Based on Sampling Data
Initially, there was some concern that the data handled in the failure prediction project might not be suitable for a data-driven hypothesis analysis. There are several thousand types of sensor data generated by each aircraft, some of which are generated as frequently as once every 0.2 seconds. Due to the huge amount of data, it was impractical to use all the past data.
After multiple discussions, dotData and JAL developed a unique model of creating input data to enable a data-driven hypothesis search using dotData. Instead of leveraging all historical sensor data, the team extracted data from a few flights before a failure had occurred as “abnormal” and some flight data from the period when the failure didn’t occur as “normal”. The combined subset of data gives dotData enough information to differentiate “normal” vs. “abnormal” states to automatically extract valuable features for predictors of failure.
“We will continue to strengthen predictive maintenance by using the feature values derived from dotData.”
“We have established a flow in which, among the features that dotData extracts based on sampling data, we pick up dozens more that we judge ‘to be of engineering significance,’ and build a failure prediction model after verifying them using internal standard methodology. This way, we are not only using the tool, but also improving the best way to use it day by day, and the results are steadily improving,” says Mr.Taniuchi.
Starting in 2019, a trial feature extraction of defects was conducted using this flow, and the team succeeded in extracting effective features that indicated signs of potential failures. In addition, when dotData analyzed defects for which valid features had already been identified using hypothesis testing analysis, the same features were detected, validating dotData’s ability to find useful features. Based on these results, we began full-scale data-driven hypothesis failure prediction analysis using dotData in 2020.
Successfully Created Features to Detect Predictive Signs of Failure
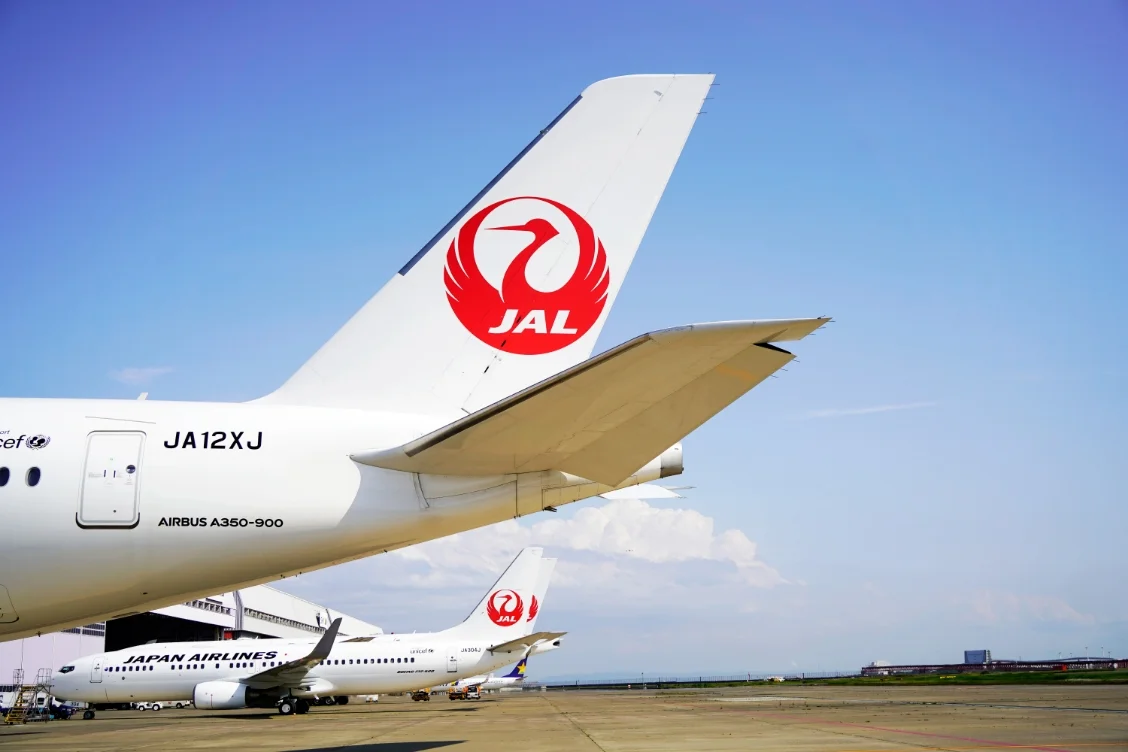
JAL succeeded in creating significant feature value through data-driven hypothesis analysis using dotData. For example, these features are effective in detecting predictive signs of failures in air conditioning system components on Boeing 787s. This effort received a special award from the Japan Aeronautical Engineers’ Association. In other case, the experience of the mechanics and engineers indicated that “ specific signals would appear while the subject system was running”, however, dotData analysis revealed features that show a specific trend while the subject system is not operational.
By combining dotData with conventional data analysis methods, the company has been able to discover new characteristics that could not be found through conventional analysis based on human knowledge, giving a major boost to the company’s failure prediction project. “There is space for improvement,”Mr. Taniuchi says, “and we would like to strengthen our efforts in both the “quality” and “quantity” of dotData utilization to achieve even better results.”
“In terms of quality, we would like to deepen discussions with dotData engineers and search for better analysis methods. In terms of quantity, we would like more people within the company to engage in analysis and predictive detection using dotData, and we have already achieved results through joint analysis with our component maintenance department.” Based on his own experience, Mr. Taniuchi offers the following advice to companies considering implementing dotData: “dotData is a powerful technology, but AI is not something you can just throw data at it and it will automatically respond to you. We recommend that you take an agile approach to clarify what you are using AI for, by running actual data and analysis and consider how to use AI in a way that is truly valuable to you.”
JAL Engineering
https://www.jalec.co.jp/Employees | 4,326 (as of April 1, 2021) |
---|---|
Established | October 1, 2009 |
About | Based out of Tokyo International Airport (Haneda), Narita International Airport, and Osaka International Airport (Itami), JAL Engineering is responsible for the maintenance, planning, and management of JAL Group aircraft, engines, and components. In addition, as a “leading company in creating the future sky” it supports safe flight operations by aircraft maintenance service for other domestic and foreign airlines that fly into airports in Japan. |