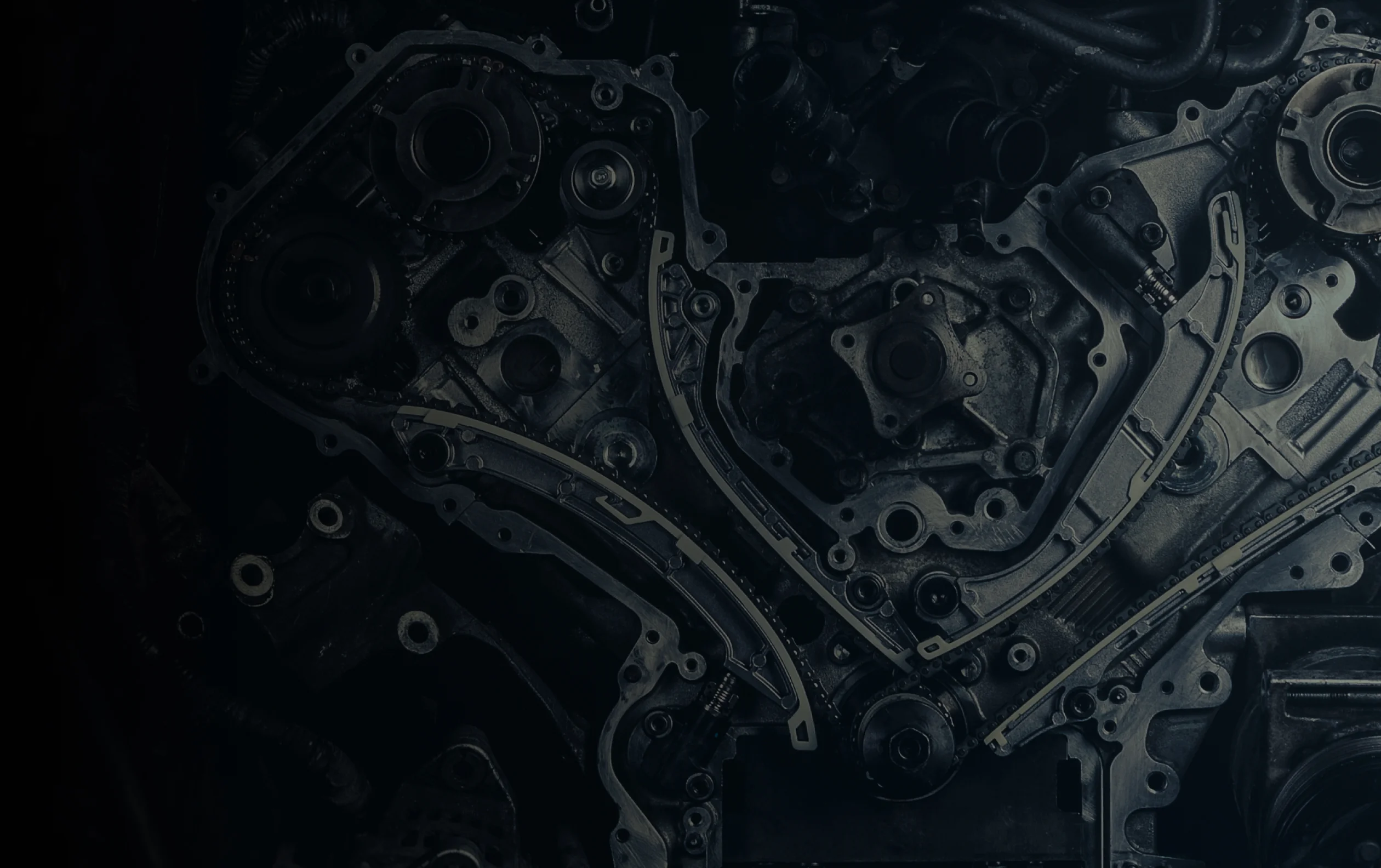
How A Global Manufacturer Lowered Inventory Costs by $10M Annually
Using AI-based ML development to leverage large data sets and improve forecast accuracy
Industry: Automotive
Solutions: Optimizing Inventory Management, Demand Forecasting
Globally, automotive companies sell tens of millions of units, with a peak of 80 million units in 2017. As with many non-perishable consumer goods, vehicles have a long shelf life, and interactions between manufacturers and consumers are not restricted to the sale.
Even after buying a vehicle, consumers need the manufacturer’s support to address maintenance and repair issues with the car. The global market for aftermarket sales was valued at over $408B in 2021 and is integral to attracting and retaining loyal customers.
A crucial part of after-sale services is ensuring consumer access to necessary replacement parts for essential maintenance and repairs. However, considering the volume of vehicles in the market and the variety of models and life cycles of parts, maintaining the needed inventory is costly and challenging. While maintaining essential inventory is critical, calculating inventory reserve needs is highly flawed.
**The market for aftermarket parts sales was valued at US$ 408B in 2021. – Grandwire Reserach
Challenges
- Manual forecasting of demand for 200,000+ parts was slow and difficult to maintain due to heavy reliance on domain experts.
- Maintaining optimal inventory levels with a large parts catalog was impossible using manually developed ML models.
- Existing ML models were largely blackbox based and provided little insight to business users.
Solutions
- dotData’s proprietary Feature Engineering (AutoFE) technology evaluated billions of data points across multiple categories – simultaneously.
- Combination of AutoFE and Automated Machine Learning (AutoML) technologies allowed the business to build models with less reliance on domain experts.
- dotData’s unique transparency provided the business with explainable insights that business users could understand.
Results
- 50% improvement in forecast accuracy based on dotData’s new model.
- $10M+ per year in cost savings due to new inventory management models.
- Greater transparency, explainability and traceability of the ML model.
Business Background and Challenges
The automobile industry faces some unique challenges in delivering after-sale services. The manufacturer must ensure clients have access to spare parts and perform aftermarket repairs even after the sale. Considering the life cycle of the products, this can be a long commitment. Automobiles are highly complex systems with a multitude of parts and components. As a result, manufacturers must maintain a stock of replacement parts. Often, this involves inventories of hundreds of thousands of components.
Because of long product life cycles and the need for long-term inventory of replacement parts, inventory management and planning can become a challenge. Some of the critical issues automobile manufacturers face include:
- Replacement parts kept in inventory can reach $100 million or more. Inventory costs are extraneous to building and maintaining enormous inventory centers.
- While ensuring replacement parts are available, maintaining a steady supply is challenging. Since life cycles vary among products, inventories have varying degrees of longevity and value lifespans. Also, the production of some parts may stop before the End of Life (EOL) of the vehicle.
Determining the appropriate inventory levels for the different replacement parts is challenging. Using AI, the company can implement demand forecasting models to model future inventory stock needs, ensuring that replacement parts are available on time while lowering the risk of maintaining huge inventories that might remain unused.
Understanding the revolutionary impact of AI-based inventory optimization in manufacturing requires comprehending the limitations of current forecasting methodologies. At present, most manufacturers perform demand forecasting based on a combination of models that are heavily dependent on domain experts, along with manual forecasting adjustments that rely on the intuition of forecasters.
This method creates two critical problems:
- There is little to no capacity to maintain and improve demand forecasting, more so when the team/person responsible for developing the method leaves the company.
- Demand forecasts are often wildly inaccurate, leaving companies with either too much or too little inventories, translating to unnecessary costs and delayed supply.
AI Solution
While using AI for inventory optimization in manufacturing provides many benefits, it is vital to understand the demand cycle of replacement and after-market parts.
- Initial demand – This is the demand that arises soon after sales. It does not require forecasting as the demand is calculated based on sales plans.
- The period from when the manufacturers stop producing the product to when its sales stop is essential – the inventory available will determine whether there’ll be a shortage, surplus, or adequate replacement parts.
- Between the end of the sales of the product model and the EOL (End of Life) – Since replacement parts are no longer in production, manufacturers often retain much inventory. Manufacturers use decaying factor models to determine the appropriate levels, such as the decaying coefficient based on peak demand.
Automobile manufacturers can leverage available data to minimize or remove the numerous blind spots associated with traditional demand forecasting methods through AI. Rather than relying on human intuition, AI-based forecasting analyzes multiple data points:
- Historical data on replacement parts
- Vehicle parts information
- Dealer information
- Production year
Along with reviewing such data, it also captures patterns to produce accurate demand forecasts, allowing manufacturers to accurately determine optimal inventory levels for over 200,000 parts.
Technical Challenges to Develop AI Solutions
While AI-based demand forecasting requires some up-front investment, long-term cost savings provide a significant return on investment (ROI), making it a must-have for automobile manufacturers. AI-based forecasting provides two critical benefits for automobile manufacturers:
1. Heavy Reliance on Domain Experts
Existing Machine Learning-based solutions are highly manual. Domain experts determine appropriate models for forecasting demand for replacement parts. Reliance on domain expertise comes with challenges. Since hundreds of thousands of parts require replacement parts, manual forecasting is tedious, time-consuming, and expensive.
Also, there’s little room to adjust forecasts based on external factors, often leading to demand forecast inaccuracies. AI and Machine Learning add automation to demand to forecast. This step alone minimizes the reliance on domain experts. By leveraging AI, inventory optimization in manufacturing can continue smoothly even after personnel and teams leave the company.
Automating the process and accounting for more data also increases the accuracy of replacement part demand forecasting, leading to cost reductions.
2. Replacement Part Demand Patterns are Becoming More Complex
In recent decades, the automobile industry has experienced many changes. For instance, with the world being more connected, car manufacturers serve large sections of the globe, adding handling repairs and providing replacement parts. Innovations such as electric vehicles and the emergence of autonomous vehicles add to the number of replacement parts manufacturers need to inventory.
These changes alone make existing ML solutions even more inadequate, enhancing the need for AI solutions to capitalize on new data sources and patterns when forecasting demand.
In addition, because demand patterns for each component vary, accounting for forecasts of over 200,000 parts becomes significantly more straightforward, faster, and more accurate with AI-based forecasting.
Why dotData?
The challenge for car manufacturers looking to capitalize on machine learning demand forecasting is finding the right provider for AI solutions. dotData helps organizations leverage the potential of AI and Machine Learning by making it simple for organizations of all sizes to use their data through fast, unique, and easy-to-use tools that build easy to interpret models.
With dotData, car manufacturers can build powerful Machine Learning models to make demand forecast easier and more efficient. More importantly, thanks to dotData’s unique approach, there’s no need for coding expertise to build custom solutions. dotData provides many benefits, but three stand out:
1. Revolutionary AutoFE Technology
dotData’s game-changing AutoFE Technology can deliver solutions that accommodate the automobile sector’s unique needs. AutoFE automatically analyzes historical data for demand, repair records, vehicle information, parts information, dealer information, production year, etc., and identifies “feature patterns” as demand signals.
dotData allows car manufacturers to incorporate new data sources for demand forecasting through its multimodal capabilities. Adding new data sources allows manufacturers to identify new patterns associated with the demand for replacement parts that would otherwise be invisible to domain experts.
For instance, based on dotData’s AutoFE, the client discovered that forecasting could be more accurate by looking at peak demand at the beginning of the product life cycle and minimum demand in the latter half of the product life cycle. Identifying the value of demand contributes to a decrease in inventory costs significantly and improves delivery efficiency.
2. Automation
As car companies continue to automate as many of their processes as possible, automating the forecasting of replacement parts becomes a logical next step. In addition to being a necessary process, it’s tedious, and any inefficiencies will directly impact profitability and customer satisfaction.
Through dotData, car companies can now reap the benefits of automation of predictive analytics in manufacturing. Moreover, it also makes it possible to quickly develop models for different parts, transforming a human-dependent process into one that requires little to no manual involvement.
3. ML Transparency
Traditional ML-based forecasts rely on so-called “black box” models, which are hard to interpret and understand. Lack of explainability often leads to mistrust of the model by business users who can’t see the reasoning behind ML-based forecasts. dotData’s technology generates easily interpretable and traceable features and models that explain how models behave, produce predictions, and what variables influenced the model. Manufacturers can build highly interpretable, transparent demand forecasting by giving insight into how the prediction models work and the reasoning behind predictions.
Outcome Highlights
After using dotData for inventory optimization in manufacturing, the company was able to experience immediate gains. The first came in a 50% reduction in forecasting error, translating to an inventory cost reduction of $10 million.
Also, dotData’s AutoFE technology automates the forecasting process, making the process no longer dependent on human involvement. Automation and Machine Learning make it possible to improve the process as manufacturers can add new data sources.
With the right AI solution, manufacturers can address one of the toughest challenges “inventory optimization,” and deliver solid, sustainable business results to survive in a competitive world.