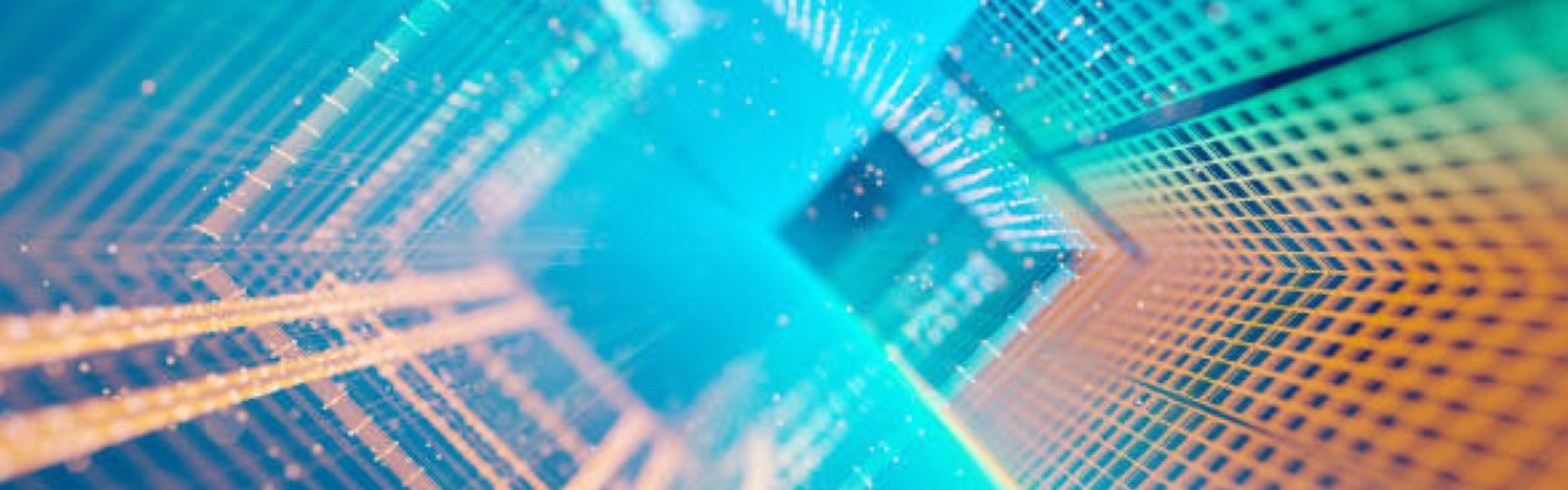
Video: How Automation Will Change Data Science
Video Transcript: How Automation Will Change Data Science
Thank you for watching this dotData webinar. I’m Ryohei, CEO, and founder of dotData. Today, I’m going to talk about a very exciting trend in machine learning and data science. How automation will change data science. Understanding deep customer behaviors and offering the right product at the right time while applying data insights to develop a new product or new services, predicting product demands over the next quarter to optimize production inventory in the supply chain, analyzing lots of IoT sensors to assess the risk of failure in energy generation plants and optimize the maintenance workflow. Data science is core to business today without any doubt, data science in machine learning is one of the most important technologies for any enterprise to innovate its businesses.
Data science is important, but it is not very easy. According to market research, 96% of organizations run into some sort of problems in AI and ML projects. There are similar statistics like 85% of big data project fails. So the question is why, why is data science or machine learning hard and fails? Lack of data scientists, lack of domain, expert communication, and expectation gaps between the business and data science teams. There might be different reasons, but the fact is many data science projects are still experiments in data science labs and they’re not, truly implemented in business.
So to understand this problem, let us review a typical data science process. It always starts from identifying critical business use cases. This is the single most important first step of any data science project. Once business use case business objective is determined. Then we have to collect the data stored in various business systems. And so we have to first collect, integrate and unify the data from different systems. Once data is unified. The next step is so-called last-mile ETL and feature engineering, relational data, transactional data, temporal data geolocational data, text data, enterprise data are so diverse and complex that this process is often the most manual and most time-consuming. There are many challenges around feature engineering. The most critical factor in feature engineering is domain expertise. Building features is nothing but extra extracting promising data patterns based on our domain knowledge. However, for a data science team or as a data scientist, it is not very easy to access deep domain knowledge. This process eventually requires a lot of iterations and interactions across interdisciplinary teams. In addition, raw data, which are not yet aggregated may contain billions of rows. We have to write a lot of SQL queries to combine aggregated tens, or even sometimes hundreds of tables with complex relationships. Overall, this data on the feature engineer process often takes months to complete.
Once a feature table is ready. It’s time to build machine learning models. There are highly accurate models like graded boosting or neural networks. On the other hand, there are many different types of models, such as a transparent model linear model or Ry. So we have to carefully select algorithms, carefully tune their parameters. And based on our understanding of project requirements, now we have features and we have machine learning models. Based on these features, we have to deploy an operational model in a production environment, quality issues, scalability issues, maintainability issues. There are many technical challenges to making data science truly work in a production environment. In summary, data is critical technology, but it is still very manual. Usually takes months to complete and requires interdisciplinary skills and knowledge that’s why many data science, uh, uh, many enterprises are still struggling to adapt data science and machine learning in their businesses. Now let’s talk about automation or how can automation help the data science team and how automation can address the programs that I described just now, as you may know, automated machine learning or simply auto ML is momentum in this community.
All AutoML automates certain steps in the data science process. It makes the data science team more efficient and more effective. We believe there are four pillars, four areas that automation helps the data science team accelerate, democratize argument, and operationalize. So let me explain each area. One by one. The first pillar is Accelerate. Data science is essentially an iteration process and it is critical to do try and alarm cycle faster for you may want to compare different machine learning algorithms or given a business problem. You can formulate it as either a regression problem or classification problem, which is better. You are provided customer segmentation information by the business team. Should do we build one model per segment, or should we build one single global model across the entire segment? You don’t know the answer until you try your ideas on actual data. So that’s why you need a lot of trial and learn cycles. Automation is a key tool to allow you to try more ideas and find better ones faster than ever before. One of doing data clients took two to three months to complete one data science project before. data. But now with. data, they are running more than 100. [Instead of ] A task in six months is more than 100, just in six months. This extremely fast turnaround to try your ideas is one of the keys to success in a data science project. And that’s why, how automation is gonna help you.
The second pillar is Democratize. Automation can eliminate the codings and deep technical knowledge such as statistics or machine learning, which are hidden behind the platform. So automation enables more people to execute data science projects. The Data scientist is an important concept in contemporary data science. As you know, data scientists are very important resources in an organization, but they are very difficult to hire and even very difficult to retain. Citizen data scientists can scale data science projects for organizations. Simply more people can execute data science processes, experienced data scientists. In this context, behave as a mentor or leader to guide a citizen data scientist. Another important aspect is democratization allow experience data scientists to focus on important tasks. There are many use cases in enterprise, but they are not equally important and not equally difficult. There are many projects that citizen data sciences can solve.
Then data science team or experienced data scientists can focus on a project with the largest business impact and bigger technical challenges. Data science democratization is not only for citizens, but it is equally important for experienced data scientists. The subtle pillar is an argument. I really like this pillar as data scientists, we usually try hundred or sometimes even thousands of feature hypotheses for a given use case based on our domain knowledge. But imagine what if we can explore millions of features, much more features than you can manually explore. Automation helps you to squeeze the data and extract the deeper business insight that complements your domain knowledge. Let me talk about a few stories. One of our airline clients usesdotData to predict cloud customers who are more likely to go to Hawaii and try to understand the customer behavior so that they can design new promotion marketing campaigns.
Data contain a lot of historical data like right history or mileage history with billions of laws. There are many interesting features produced bydotData platform. For example, there are a couple of features related to gender. The first one is that male customer is much less likely to go to Hawaii. This is a very well-known fact. So there is no surprise or, and airline customer. The second feature was made to customers who have had more flight Lakers in the last six weeks. Uh, even much less likely to go to Hawaii, many flight Lakers in six weeks. This may be a very business person who is traveling a lot for a business trip. So that type of person has no time to take the family to Hawaii.
The airline customer may be able to design some new promotion campaign for a customer who has this type of feature like, oh, Hey look, you are too busy. Why don’t you take some vacation and take your family to Hawaii and enjoy your summer? So this type of knowledge is bringing a lot of new ideas for the use of automation platforms and delivers a lot of business insights. I want to emphasize that this hypothesis were automatically produced by machine without any human intervention. So automation has huge potential to discover many valuable insights that you have never imagined before. In that argument, you were domain expertise.
The last pillar is Operationalization. Data science projects truly generate business value. Not when we build the model. It generates value when the model is operated in business. However, there are many challenges to deploy on operationalizing data science in business. Often time data science lab environments in the production environment are very different. Also, the data science team typically works with partial or downsample the data for validation, purpose, or data scientists are not software engineers. So there are many lead implementation efforts required for productionized data science process. In this context, automation automatically produces production, ready calls or APIs that eliminate a lot of these challenges, a quality issue, scalability issue, or, or maintainability issues, and then significantly lowers the barrier to bring out your data science project from labs to business.
I believe that this last area operationalization of data science is becoming more and more important. Given model development itself is becoming easier with excellent tools, including auto. Again, data science can make a real business impact when it is integrated into the business system and the business processes and used in day-to-day business automation helps you to operationalize data science. I hope my presentation software gives you an idea of how automation is going to help your organization for the successful data science journey. And here, let me briefly explain our offering asdotData thought data is a data science platform, which supports full-cycle data science processes from raw data through data in feature engineering to machine learning in production. The most unique component is AI power. In the feature engineering, you put tables, temp, table, 50 tables. As many as you want, then our algorithm exports, millions of feature hypothesis, and find out relevant and promising feature patterns for giving use cases.
We also have the auto component, which exports hundreds of state-of-the-art machine learning algorithms in tune with their hyper permitter for the best accuracy. The Tanner on time withdotData is significantly reassurance previously. It was months withoutdotData, but now we are talking about the days to complete this end-to-end process dotData season data scientists can perform this complex process, which makes your data science project scalable and sustainable AI power feature engineering delivers lots of interesting and important business insights to augment your business knowledge and the production-ready pipeline is immediately exposed as API, which makes you very easy to productionalize your data science project.
This is the last slide of this presentation in May 2020, uh, 2019, one of the top market research from Forester published a new wave port automation, focused machine learning solutions and dot nano data named, uh, a leader, in particular, our ability to automate data and the feature engineering process beyond standard AutoML was highly respected. Foresta published a label specifically focused on AutoML. This indicates that automation is going to be the next key technology in machine learning and in-depth enterprise data science. I hope this webinar helps you to understand the value of automation in enterprise data science and how automation will change data science, please, to our website, wwwdotData.com to learn more. Thank you again for watching the webinar.