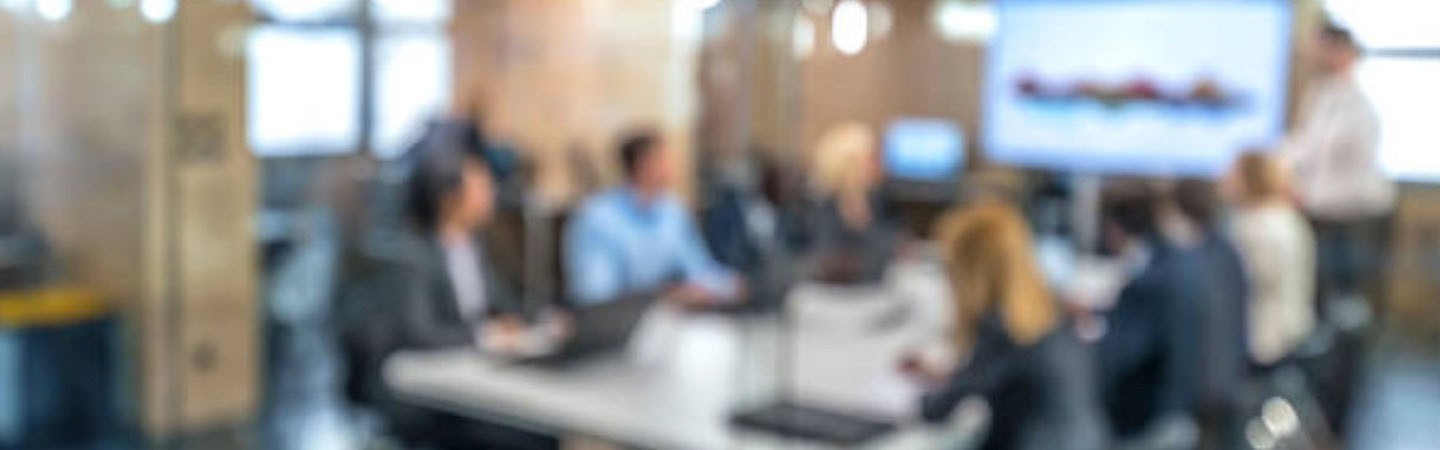
Does The Popularity of AutoML Mean the End of Data Scientist Era?
McKinsey Analytics wrote an article on the evolution of automated machine learning (AutoML) titled “Rethinking AI talent strategy as AutoML comes of Age.” McKinsey argues that the growing popularity of AutoML tools drives a radical new way of thinking about data science talent. By automating the data science process, AutoML platforms expand the reach of users to include business experts with extensive domain knowledge, non-data scientists, and operational experts. The key takeaway is that companies are best served by not putting all their resources into the fight for sparse technical data science talent but should instead focus at least part of their attention on building up their troop of AutoML practitioners, who will become a substantial proportion of the talent pool for the next decade. CIOs, data science, and analytics leaders will have to rethink their AI talent strategy fundamentally. The Covid-19 pandemic, budget cuts, and the pressure to do more with fewer resources are further adding fuel to this fire – the AutoML revolution is here to stay.
Why is the interest in AutoML technologies exploding? What are the implications for organizations, especially small and midsized businesses? What’s in store for data science professionals?
The AI-driven intelligence revolution has been gathering momentum worldwide, with large organizations experimenting with AI and ML for quite some time. There has been a strong wave in the industry to build AI-driven predictive applications from predicting financial fraud, reducing unplanned downtime, and forecasting demand. That implied hiring an army of data scientists. A LinkedIn survey from July 2018 reported a shortage of about 150,000 data scientists in the US. As organizations were moving forward with digital transformations, powered by data science and machine learning, implementing data science into various aspects of their businesses was proving difficult. Also, the vast majority of data science opportunities were with large organizations. Small and midsized companies lacked the data infrastructure and did not have the financial muscle to hire a legion of data scientists. Another critical challenge was the sheer amount of time needed to complete AI and ML projects, usually in months, and the incredible lack of qualified talent available to handle such projects, managing data pipelines and putting models in production.
Given the variety of data science projects and the substantial amount of data manipulation required, building and retaining this team is an arduous task. AutoML addresses some of the biggest challenges of data science – automating the laborious, iterative necessary steps in building machine learning models, eliminating human errors, and reducing the time it takes to construct production-ready models.
Data science projects require an interdisciplinary team of data scientists, ML engineers, software architects, BI analysts, and subject matter experts. As the following illustration of a data science workflow shows, data scientists spend most of their time in data preparation, modeling, and parameter tuning. The advent of AutoML tools has changed the mix of talent needed to execute data science projects.
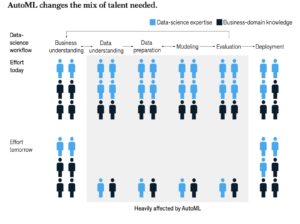
The first-generation AutoML platforms, aka AutoML 1.0, were designed to build and validate models automatically. While still being used today, these traditional platforms automate only the machine learning component of the process but do not address data preparation and feature engineering, the holy grail of data science. The next-generation platforms, aka AutoML 2.0, include end-to-end automation from data preparation, feature engineering to building and deploying models in production. These new platforms help development teams reduce the time required to develop and deploy ML models from months to days. AutoML 2.0 platforms address hundreds of use cases and dramatically accelerate enterprise AI initiatives by making AI/ML development accessible to BI developers and data engineers while also accelerating data scientists’ work.
By using Full Cycle Data Science Automation, enterprises don’t have to invest in as many skilled data scientists or teams of engineers for each project. AutoML 2.0 also empowers the so-called “citizen” data scientists bringing AI to the masses. Interpretable features help organizations stay accountable for their data-driven decisions and meet regulatory compliance requirements. This allows domain-experts to interpret models more quickly, especially with transparent outcomes, increasing the process’s effectiveness and efficiency. This “democratization” of AI provides a unique opportunity for enterprises of all sizes to integrate machine learning into business applications with the shortest time-to-market. Empowering existing BI and data professionals enables businesses to eliminate data science dependency and address the digital transformation roadblocks in terms of costs, ROI, and scalability. By augmenting BI with AI, even smaller businesses can reduce operating costs, improve quality, reduce churn, and generate new revenue streams.
So what does that mean for the future of data scientists? The thought that AutoML will bring the end of data scientists as a profession is exaggerated. Data scientists will always be in demand to handle very complex, unique use cases that are mission-critical or require very high accuracy at the expense of interpretability. Mckinsey team is spot on in their analysis that purely technical data scientists will still be needed over the long term, but simply far fewer than most currently predict. Analytics experts estimate that demand for AutoML practitioners is likely to be twice as high as demand for data scientists as companies build out their talent strategies with both levels of expertise over the next five years.