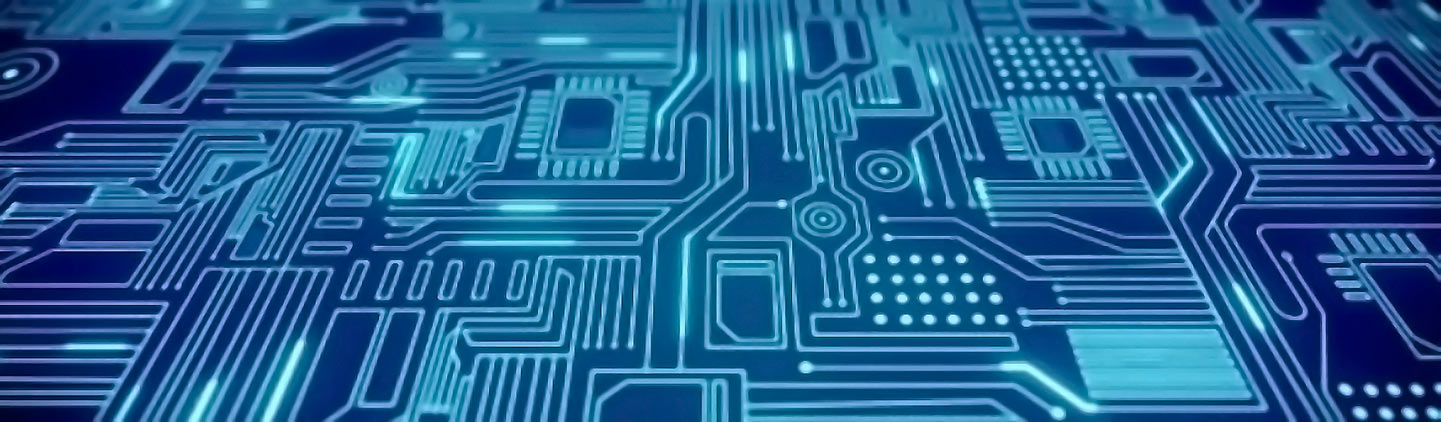
The Evolution of Analytics, BI, and the next big thing in AI
Why automating feature discovery and engineering will be a game-changer for enterprise AI
Updated August 3, 2022
Data Everywhere
Large enterprises have long known that data is at the heart of rapid decision-making and better long-term organizational health. Most organizations’ challenge is not deciding if leveraging data for decision-making is useful but how to do it. Anyone who’s been around the BI industry long enough knows that for years the big goal was “self-service BI.” The idea was that business users would somehow become dashboard mavens who could easily bypass and displace business analysts and developers to flood the enterprise with dashboards. Platforms have increased in complexity, and the reality is that analytics is still primarily performed by skilled analysts with in-depth knowledge of data management and visualization techniques. Fast forward to 2021, and the same conversation can be had about AI and Machine Learning – and how they will impact the world of business intelligence.
Prediction vs. Cognition
When BI folks speak of AI and Machine Learning, they often describe the underlying technology needed to power predictive analytics. Predictive analytics is not a new concept in the world of BI. It has existed in some form or another for decades. In layman’s terms, Predictive Analytics predicts what “will” happen. The next logical evolution is to provide recommendations for the best course of action. Gartner describes this phase as “prescriptive” analytics, while Deloitte in 2014 defined it as “Cognitive” analytics. While the debate goes on about terminology and actual use-cases, the overall consensus is always the same – with the advancement of computing power, we will be able to apply more sophisticated mathematical algorithms to our data to create systems that, more closely, resemble how a human brain interprets data to aid in decision-making and planning. In some respects, the combination of business intelligence and AI and ML will begin to finally deliver on the promise of the decision-support systems of the 1980s.
The AI Challenge: It’s Hard
Any way you describe it, the challenge for most enterprises in delivering on the promise of prescriptive analytics or cognitive analytics is that developing the necessary ML algorithms is time-consuming, resource-intensive, and prone to error. The challenge with AI appears in the latest data on AI adoption in the enterprise. In a 2021 survey conducted by Rackspace Technologies Inc, 1,870 organizations across various industries showed that only 20% had what they considered a mature ML strategy. Even in 2022, practicing enterprise-wide AI and Machine learning can be challenging. According to an IDC survey, while 31% of organizations have AI in production only one third of those claim to have reached a mature state of adoption. Lack of production-ready data and appropriate talent were organizations’ most prominent challenges in developing a well-established and mature ML strategy. The same IDC survey found that dealing with data was the biggest hurdle organizations faced as they invested in AI infrastructure. Mirroring the “self-service BI” push, enterprises have tried to adopt automation as a critical technology to automate as much of the process as possible to mitigate the cost and sparse availability of deep AI/ML talent. Just like in the world of BI, the reality is beginning to settle: there is no “one-product” approach for everyone. While smaller organizations will most definitely benefit from end-to-end automation of the AI/ML process. However, the sheer complexity, size, and scope of large-enterprise projects make this unlikely.
Assisted Automation for Predictive Analytics
Large enterprises tend to have well-established and proficient data science teams that are very proud of their work developing AI/ML models for their business. However, the challenge for these larger companies is that even with all the in-house talent, AI/ML models often take too long to complete and often lead to problematic deployments. One of the biggest challenges for any large-scale data science organization is the inherent cyclical nature of AI/ML development. The problem is that even sophisticated AutoML platforms require flat data tables as the source input to evaluate algorithms and optimize parameters. While AutoML has been able to accelerate training ML models, the hardest part of the job – building the flat table required to begin with – is still a mostly manual process. While developers and data scientists gain an inherent “gut feel” for which columns of data might yield the best results, this is limiting since the “hypotheses” that are the basis of selecting the columns of data for the feature table cannot be tested until the model has been thoroughly evaluated. AI’s manual nature can lead to repetition and lengthy iterative cycles where experiments are required to discover useful new features.
Automating Feature Discovery and Engineering: The Next Step
Automated feature engineering is not new. Most AutoML platforms perform some form of analysis of the source flat table to infer new features using mathematical derivatives. That, however, only solves a very small part of the problem. The more significant challenge is knowing which tables and columns from your data lake or warehouse might be useful until you’ve tried them. It’s discovering these “unknown unknowns” that creates the endless “hypothesize, test, repeat” cycle for larger organizations. However, new platforms are on the horizon that can help established, mature data science teams address this problem. By leveraging AI’s power, these new platforms can act as an accelerator for the data science team – giving them ways of rapidly prototyping scenarios without spending weeks or months building flat tables – only to start over. These new generation automation tools can also provide a quick way of augmenting the work done manually by the data science team to help derive new and powerful models based on retained knowledge and discovery.
Self-service BI was not a failure; it needed to find its place in the BI ecosystem. Rather than creating armies of Line of Business Users building dashboards, self-service BI enabled a new generation of power users who took some of the analytics teams’ workloads, giving them the freedom to concentrate on more critical projects. Similarly, feature engineering platforms will not displace the data scientists but provide them with powerful new tools to accelerate their work and build better models that are more likely to succeed.
Share On