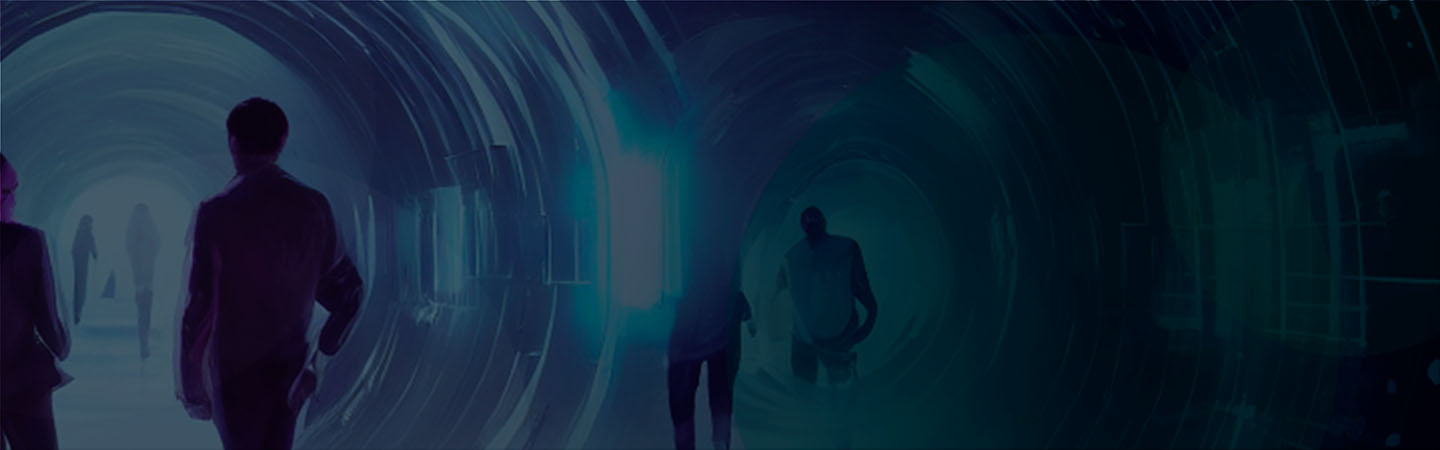
6 Predictive Analytics Use Cases In Advertising
6 key ways advertisers can benefit from predictive analytics and how to get started
Predictive Analytics, in its simplest definition, is just the process of using historical data to detect patterns and build predictions for future outcomes based on similar patterns repeating in the future. In advertising, this might include examples like using historical performance data from digital advertising campaigns on Google to build predictions of how similar campaigns might perform in the future. Unlike other forms of analytics – like dashboarding and Business Intelligence (BI) – also known as “descriptive analytics,” predictive analytics relies on mathematical algorithms to predict future outcomes. It’s a powerful tool for optimizing advertising spend.
Why Predictive Analytics in Advertising?
Predictive analytics, driven by Machine Learning, can be a powerful tool for data-heavy industries. The world of advertising, especially digital advertising, relies heavily on data but has recently been subjected to seismic shifts due to increased privacy concerns. Apple’s decision to increase privacy in its IOs operating system has caused a ripple effect on the advertising industry, which has long relied on cookies and tracking to create optimal campaigns and to boost performance by keeping close tabs on the daily patterns of consumer behaviors.
While the cookie-less world is the new reality for advertisers, predictive analytics can still be a powerful tool, allowing advertisers to use their wealth of data with machine learning techniques to make informed decisions about their advertising campaigns. There are several potential use cases for predictive analytics in advertising:
- Targeted advertising
- Ad optimization
- Cookieless advertising
- Customer lifetime value prediction
- Customer segmentation
- Predictive maintenance
6 Use Cases for Predictive Analytics in Advertising
Targeted advertising:
Precision targeting is at the heart of advertising. Predictive analytics can leverage the wealth of data that most advertisers have on their target consumers to build predictive models based on demographic, psychographic, and other factors. By leveraging Machine Learning, advertisers can build precise targeting criteria to optimize ad buys and focus campaigns that ultimately drive higher return on ad spend (ROAS).
Real-time Ad optimization
Real-time ad campaign optimization is possible using machine learning and predictive analytics. Advertisers can improve ad targeting, placement, creative and other factors by using historical data to predict how specific ads will perform based on multiple criteria. This is especially significant in high-volume advertising channels like digital advertising, where optimizing campaigns on the fly can significantly impact ad performance.
Cookieless Advertising
Relying on tracking cookies and pixels has been the mainstay of digital advertising for more than two decades. With increased focus and scrutiny on privacy and consumer protections, digital advertisers need to turn to new techniques. By leveraging the wealth of in-house customer data – like point-of-sale information (POS) – advertisers can build new predictive models that can substitute cookies to provide highly targeted advertising opportunities while still providing the degree of privacy consumers demand.
Customer lifetime value prediction
Predicting the lifetime value of a customer (CLV) can help advertisers allocate budgets more effectively to maximize customer value. By combining CLV with other predictive techniques, advertisers can also explore means of increasing the lifetime value of specific segments that may originally have been predicted to perform poorly or can provide greater clarity on new customer segments and targeting to increase the volume of high-CLV clients.
Customer segmentation
Bucketing customers by key characteristics based on their likelihood of responding to specific ad types, creatives, channels, etc., can boost ROAS while creating a better shopping experience for consumers. By leveraging predictive analytics and machine learning, advertisers can tailor campaigns based on specific segments and continually revise and adjust customer segmentation characteristics to optimize campaign performance on an ongoing basis.
Predictive maintenance
While predictive maintenance is typically associated with manufacturing use cases, advertisers can leverage the same techniques to model equipment or software failures for planned campaigns. Whether the campaigns are digital or physical, predicting downtime of specific ad platforms and vehicles can reduce costs by minimizing downtime and ensuring that campaigns run uninterrupted to maximize return.
What You Will Need to Succeed
Get buy-in from the business
It may seem odd starting from a non-technical point, but lacking buy-in is one of the biggest failure points for any predictive analytics project. Whether it’s from executives, who are not convinced of the value or the likelihood of success, technical staff that may be unprepared for the work needed, or a combination of reasons, ensuring that you have top-down agreement on the need for predictive analytics to boost your advertising success is critical. It may also help to start small, with a specific goal to prove the strategy before expanding.
Define your problem
The second step is defining your business problem. What are you specifically trying to predict? How will you measure whether the model has succeeded or failed? Increasing ROAS might be tempting, but it’s probably too broad. Try to think of metrics associated with your test’s success or failure. For example, If you want to leverage in-house Point of Sale data to target campaigns more accurately, you might want to measure the impact of a coupon campaign on sales. You need to identify how you will measure sales success (unit volume? Revenue? both?) and over what period you would want to measure baseline performance before running an optimized campaign.
Identify the right data
With your problem identified, the next step is mapping out the needed data set. In our example, we would want to source as much PoS data as possible. Still, it would need to be augmented with client identification data – to replace the cookies traditionally associated with a customer-targeting campaign. This “ID-PoS” type of data is commonly available in the world of retail (for example) and will need to be made available to your analytics team, cleansed, prepped, and massaged so that it’s Predictive Analytics “ready.”
Loop in the SMEs
There are many technical steps between getting your data ready and building models, but you will need to loop in your subject-matter experts at some point. The people in the organization are best suited to identifying which data columns in your data might be most valuable in building an effective model. This part of the process can be very time-consuming and costly if you don’t have a method for accelerating the process. [link to page]
Create and identify the best model
With your data selected, your targets declared, and ready to be measured, you are now ready to proceed to the next step – building the ML model you will need to predict your outcomes. Creating many different ML models will provide different insights into the predictive power of your data and offer choices in prediction accuracy, speed of prediction, and ease of explaining predictions to others. Once again, having platforms that can automate the process can be immensely useful.
Deploy the model
The last step in the process is deploying the model. However, the critical part of this step is also to provide a means for monitoring and retraining the model as your data changes over time. Your model will likely lose accuracy as your real-world data drifts over time or consumer behavior changes. Monitoring model performance or “drift” and providing new data to retrain it are key steps to maintaining high accuracy.
Conclusion
This introduction to predictive analytics use cases for advertising is just the first step in understanding the power of machine learning and how it can be used to boost advertising success. As economic uncertainty increases and new pressures from increased privacy make success in advertising less certain, bringing new tools and techniques on board to boost the success of campaigns can provide significant benefits in a very short period.
Share On