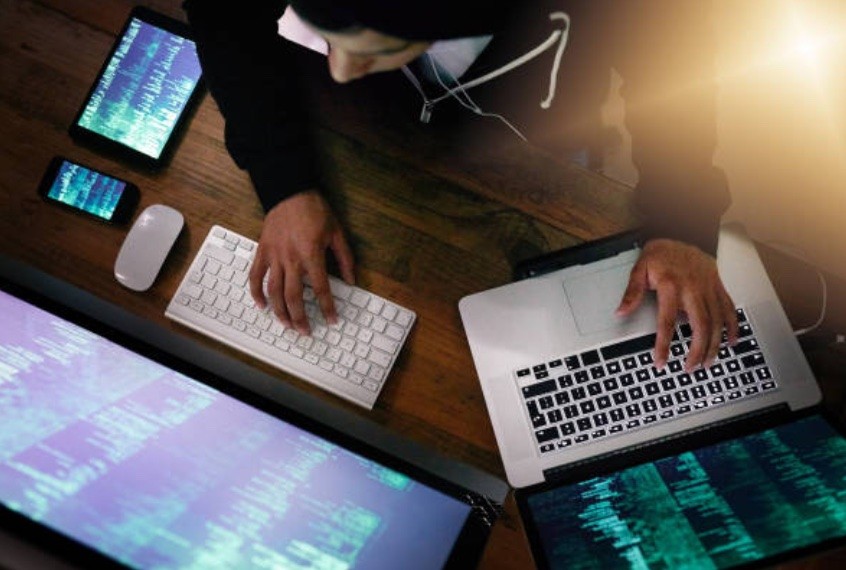
What Is Predictive Analytics and How To Get Started
TL: DR: Predictive Analytics is using historical and real-time data to generate useful insights and predicting critical outcomes in the future. A large number of organizations are leveraging this AI-powered technique to reduce risks, improve operations, cut business costs, and increase the bottom line.
What is Predictive Analytics?
Gartner defines Predictive Analytics (PA) as a form of advanced analytics which examines data or content to answer the question “What is going to happen?” or more precisely, “What is likely to happen?”, and is characterized by techniques such as regression analysis, multivariate statistics, pattern matching, predictive modeling, and forecasting. Grandview research recently estimated that the global market for predictive analytics is growing at a CAGR of 23.2% and projected to grow to $23.9 Billion by 2025. Initially, the purview of a few visionary companies, predictive analytics is rapidly gathering momentum in the market. Several industries such as banking, financial services, insurance, and manufacturing are using predictive analytics to forecast demand, prevent customer churn, and perform anomaly detection for predictive maintenance.
According to this Forbes article on the state of AI and ML, advanced algorithms and predictive analytics are among the highest-priority projects for enterprises adopting AI and machine learning in 2019. As this illustration from Dresner Advisory Services shows, predictive analytics is a top technology initiative for business intelligence (BI). Since reporting, dashboards, data integration, and visualization are mature BI capabilities, augmenting BI with AI for predictive modeling is becoming critically important.
The focus of BI has been analyzing historical performance – what happened in the past and to an extent what can be done to prevent a recurrence using traditional analytics. Predictive analytics, on the other hand, offers the ability to look into the future and enable organizations to take appropriate actions. It can enable organizations to solve very complex business problems, manage risks, and identify new opportunities. Predictive analytics is ideal for solving complex multivariate problems such as IoT time-series data or situations where traditional approaches such as classical statistical techniques cannot be applied.
For example, timely predictions can identify financial fraud, predict buyer behavior, enable dynamic pricing, or detect a faulty component from going into a final product. By providing real-time dashboards, predictive analytics can improve the performance of BI platforms. Due to advances in cloud computing infrastructure and technological breakthroughs in AutoML platforms (that hide all the technical complexities with AI-Powered Automation), predictive analytics is very much affordable for even small and medium businesses without huge analytics or IT teams.
Types of Predictive Models
There are five main predictive analytics models used by data scientists: Classification, regression, time series, clustering, and anomaly detection. Patterns discovered by predictive analytics models can predict future behavior. Some predictive analytics models are easier to understand than others, but they all have their place in business and data science.
Common Use Cases for Predictive Analytics
Predictive analytics has a number of valuable applications across many industries and for a wide range of use cases.
In banking, predictive analytics is leveraged by implementing processes and procedures that inform the bank of potential risks and fraud. Many industries also use predictive analytics for customer segmentation, policy optimization, churn prevention and more. Read our blog post that summarizes many more predictive analytics use cases by industry.
Predictive vs. Prescriptive Analytics
You may have heard these terms used interchangeably, but there are some key differences. Predictive analytics helps us predict future outcomes, whereas prescriptive analytics takes those predictions to the next level by identifying the likely outcomes of these predictions and suggesting ways to alter them.
Predictive analytics is understanding what will happen, and prescriptive analytics is understanding how to make it happen, prevent it, or change it.
Get Started with Predictive Analytics Using AI and Machine Learning
Organizations must leverage predictive analytics, an advanced form of analytics, and data science automation to gain greater agility and faster, more accurate decision-making. The best way to get started with predictive analytics is to use automated machine learning (AutoML) tools. A key hurdle in analytics and AI/ML has been finding talent – data scientists, data architects, machine learning engineers skilled in building, testing, and deploying ML models. That’s where automation can play a big role. AutoML platforms allow non-data scientists to automatically build, validate, and deploy predictive models at the touch of a button. The new breed of AI automation platform empowers BI professionals to leverage their data skills and bring predictive analytics to BI stack.
However, those organizations that don’t have any analytics capability or in-house data science expertise will require more than just an AutoML platform. The software platform needs to be bundled with training, use-case co-development, as well as a fully-managed SaaS environment as a predictive analytics program. A dedicated program will be ideal for companies with a strong BI practice and that wish to add predictive analytics capabilities without adding or growing expensive resources and data science teams. The BI and analytics leaders at these companies may have prioritized predictive analytics use cases but either don’t have a budget for hiring more data scientists or simply don’t have in-house data science skills. CIOs, BI, and analytics leaders should look for the following features to accelerate the predictive analytics journey:
- AI-Focused data preparation that includes data value cleansing, statistical data profiling, and data re-architecting
- AI-Powered Feature Engineering with feature hypothesis, query generation, and feature relevance validation
- Model Production with prediction and retraining endpoint generation, model package, and containerized model generation.
The goal of a predictive analytics program should be to leverage existing resources, in-house BI teams, data engineers, or business analysts, and allow them to add more value with AI + BI quickly. Predictive analytics is bringing exciting new opportunities to digital-ready enterprises. A fast start program that is designed to provide everything organizations need to get started with AI is the best approach to incorporate predictive analytics in business applications.
Share On