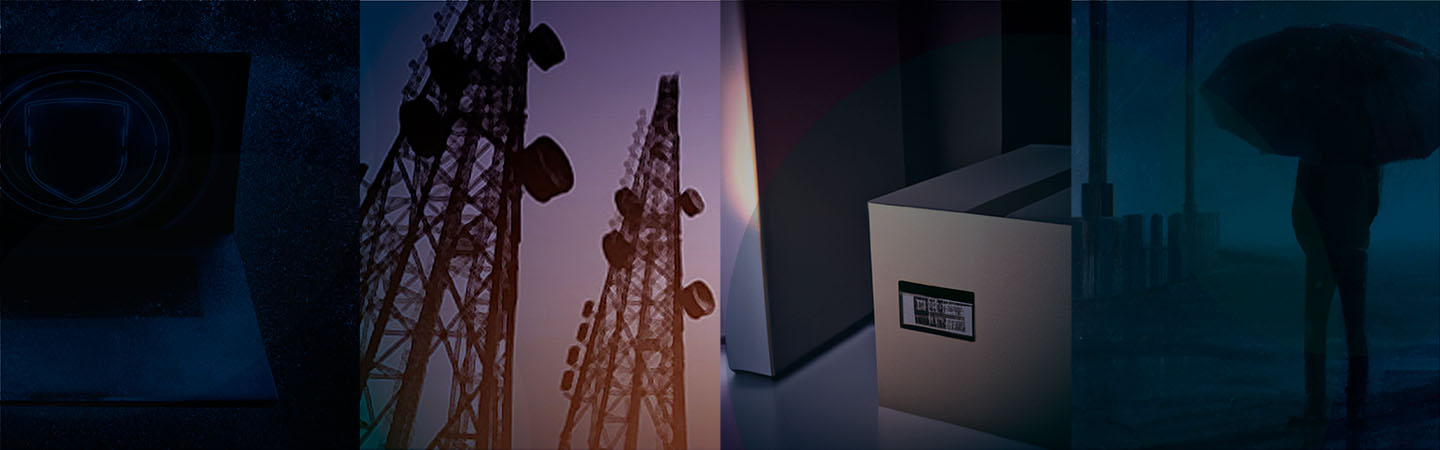
Predictive Analytics Use Cases by Industry
- Thought Leadership
Predictive analytics is a form of advanced analytics that can help us predict future outcomes and identify risks and opportunities. It can consist of predicting customers’ behavior, future market trends, potential risks for our business, profits, and opportunities. Predictive analytics uses machine learning and statistics to analyze historical data and make predictions.
Predictive analytics has a number of useful applications in many industries and for a wide range of use cases. Let’s explore some examples of predictive analytics applications in the banking, manufacturing, retail, insurance, and telecom industries.
Banks can leverage predictive analytics by implementing processes and procedures that inform them of potential risks and fraud. A straightforward example is that the bank will track the customers’ spending habits together with the location; therefore, when an unusual activity that stands out from the pattern we have for this customer appears in the systems, both the bank and the customer can be alerted. This way, the bank can quickly check whether it is indeed the customer using their card to protect its customers promptly. Another way banks use predictive analytics to detect fraud is by analyzing all of the transactions to see if there is anything that stands out that would not be expected based on the historical data (e.g., a large number of total transactions, large sums transferred, etc.).
Customer lifetime value is a measure that predicts the total income the business can expect from a given customer. Predicting this value can help us decide how to navigate a relationship with the customer. This can lead to better optimization of resources so that our attention is focused on those customers that will bring in more profits to our business. It can also lead to a reevaluation of the services we offer and a discussion with the customer to understand what we can do to raise the customer’s lifetime value.
Manufacturers can leverage predictive analytics by establishing predictive maintenance. Data collected from the equipment and data about the equipment can predict when maintenance will be needed. This gives managers more time for better planning and allocating of resources, equipment, and workers’ time. The more data we have, the more accurate the predictions will be. This data can predict when maintenance will be needed and warn us about potential unexpected breakdowns.
Manufacturers can also use predictive analytics to set up alerts that would inform them about potential quality issues. This allows the manager more time to change the processes in place to allow for better performance. Inspections can then be done when needed, and any issues will be caught on time.
Cross-selling works by understanding which additional products the customer is likely to buy based on the analysis. Up-selling is adding more features to the current product or offering a higher level product for a bigger profit. In retail, this can consist of analyzing the customer’s profile and shopping and browsing history and, based on this, predicting what else we can offer to the customer. This can be subtle but can result in increased sales.
Customer segmentation is the process of dividing customers into several groups based on their characteristics. Such a division can help us get more insights into our customers. For example, this can result in three different groups with some basic characteristics: young, impulsive customers who are always looking for the latest trends, customers who appreciate organic and eco products, and budget customers who always look for the latest sales. Once we have such a division, we can change our marketing strategy to target each group individually. By doing this, we can appeal to their interests precisely and lead them to products they are more likely to find interesting, and in turn, this will result in higher sales numbers.
As previously mentioned in retail, customer segmentation works the same way in insurance. One important reason why insurance companies need customer segmentation is to help them build stronger relationships with clients. Understanding your client can help you nurture the relationship properly. It helps the business know what services to offer and when is the most suitable time to do so.
Numerous insurance companies are already using predictive analytics to tap into customer data to create personalized policies. Offering personalized services helps increase customer satisfaction. This makes it difficult to beat the competition without offering personalized policies. By targeting different groups, we can make policies that decrease risks and increase profits.
Cross-selling in telecom often works in a way that, together with one service, they will offer others they have. If a customer buys mobile internet, they will very often offer to sell a mobile phone as well. Even though both are existent, up-selling is way more common in telecom. A customer will almost always be offered a package with more features than the one they are going for but is at the same time more expensive. However, predictive analytics helps to tell us exactly what kind of features we need to add to a given package so that the new, higher price will be acceptable for the customer.
Churn prediction helps us detect which customers are likely to leave our business, for example, cancel the subscription to our services. Companies usually have some incentives, offered only when the client informs the business that they want to cancel the subscription. While it works to keep a small percentage of clients who would have otherwise left, it is far from the best way. An efficient way is to combine predictive and prescriptive analytics to understand why clients want to leave. Before they even decide to leave, we can offer to make changes in their subscription to keep them.
As we have seen, across multiple industries, predictive analytics can provide significant benefits through multiple industry-specific use cases and applications. There are, however, some commonalities between the uses of predictive analytics that make it applicable to just about any industry:
Many companies already use predictive analytics, and regardless of the business your company is in, it is highly likely that your competitors are already employing predictive analytics in one way or the other. Predictive analytics gives businesses an edge that is difficult to gain with anything else. Using the power of machine learning and statistics to see the future and guide your business through it with intelligent decisions is the only way to stay ahead of the competition.
Using predictive analytics, we can anticipate the needs and expectations of our customers. We can use it to create hyper-personalized marketing, which enables us to serve each customer precisely with the products they are looking for with the message that will draw their attention. We can use predictive analytics to detect precursors to changes in customers’ behavior, allowing us to be more proactive and serve those needs before they are even fully established, especially before the customer explicitly communicates them.
Predictive analytics can help with both analyzing the leads as well as the existing customers. Using predictive analytics systems, salespeople can get better scoring and a better idea of how likely the lead is to become a customer. In cases where we have several leads we want to pursue in a short period of time, this can give us a better idea of where to focus our attention. We can also manage our customers in a better way by anticipating their needs before they are even aware of them. This helps us make such decisions that will prevent them from leaving.
Risk management is important in any business. Risk management is the process of identifying the risks, quantifying them according to the level of impact they could have on our business, and developing a strategy to mitigate them. Utilizing predictive analytics to analyze all potential risks and threats makes it easier for us to manage them. Once patterns are discovered, we can move on to developing a strategy for their mitigation. With predictive analytics, we can explore the effect that different strategies would have and then select the one that gives the best results.
If you are just getting started with predictive analytics, dotData’s unique combination of powerful software and deep industry expertise can provide you with an accelerated way of onboarding your first use case for predictive analytics. dotData’s feature factory-based products allow you to build predictive models directly from raw enterprise data, skipping the tedious and time-consuming process of identifying which tables and columns to use, what data relationships to experiment with, and which machine learning algorithms to use for your predictive model.