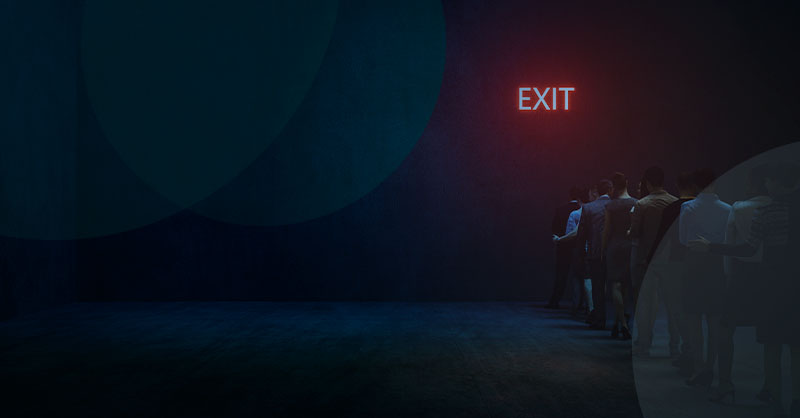
How to Improve Customer Targeting to Increase Cross-Selling
Case Study: Increasing Cross-Selling Conversion Rates Tenfold
Challenge
A major telecommunications company needed to identify customers most likely to upgrade services. Their legacy methodology yielded a conversion rate of only 1%.
Solution
dotData’s AI-powered feature discovery and evaluation platform identified correlated behaviors that were not previously obvious or easily discovered
Results
With dotData’s platform, the client was able to increase the conversion rate by 1,000% and delivered precise targeting criteria for marketing campaigns
In 2021, the global market for mobile payments exceeded US$ 1.78 trillion. Accelerated by the worldwide pandemic, customers have been increasingly keen to find alternative, cashless, contactless payment methods. A Research and Markets study predicts that this trend will accelerate, with a CAGR of 22% from 2022 and a total market value of US $6 trillion by 2027.
As global network infrastructure sees additional investment and expansion, customers respond by adopting mobile payment platforms as a regular go-to solution for payments on the move. According to Juniper Research, over two billion people currently use mobile payment technology, which is expected to swell to more than 4 billion by 2025.
Seizing the opportunity of this booming technology, telecom companies have been developing proprietary mobile payment services in fierce competition with one another. Positioning this new service as a valuable add-on, telecommunication providers achieve higher revenues while building customer loyalty and reducing churn.
However, mobile payment is a competitive field. Telecom companies compete with one another to offer this service, and they also have established banks, software giants, social media platforms, and tech start-ups as entrenched competitors. Some best-known providers include Apple Pay, PayPal, Stripe, Google, and Facebook Pay. Retaining a competitive edge in such a crowded marketplace can be highly challenging.
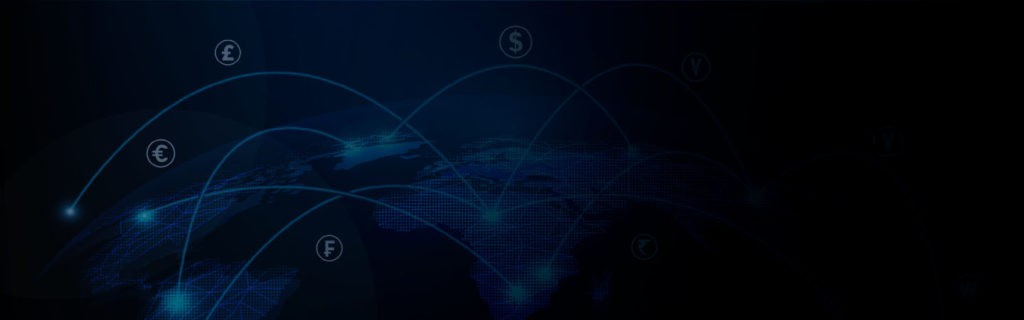
The Challenges of Mobile Payment Provision
For telecom providers, mobile payments are a new product. They don’t have a wealth of experience in the field or the necessary depth of insight into customer preferences and habits within this sector. Such comparative newcomer providers have difficulty identifying which of their millions of customers are most likely to adopt such a product, how they will use it, and what form a mobile payment platform should take.
To increase sign-ups, telecom companies need to build target customers by identifying key consumer behavioral patterns that indicate the likely adoption of target marketing campaigns for these new prospects.
Finally, services need to provide attractive incentives for early adopters compared to competing products.
The Client’s Dilemma: Too Much Undifferentiated Data
Telecommunication providers aren’t short of customer data. They have billions of data points to sift through. The problem, therefore, is where to start. The challenge is to discern behavior patterns in the data that indicate regular mobile payment usage and the likelihood of these customers signing up for the new service.
dotData’s client had achieved ten million mobile payment service subscribers yet also had a much larger pool of phone service customers who weren’t using the mobile payment option. The client’s current conversion rate, across all service users, was less than 1%. They neither knew which data to focus their attention on nor how to extract the needed behavioral information.
Furthermore, the client didn’t know which specific behavior would correlate with potential conversion to the mobile payment service. Therefore, their current marketing strategies were extremely hit-or-miss, and their sales strategy involved using a call center to run through an undifferentiated list of current customers, resulting in very few conversions.
dotData: An AI-Powered Solution to Increase Cross-Selling Opportunities with Improved Customer Targeting
dotData was called upon to find AI-driven methods for improving the client’s customer targeting regarding the company’s new payment app. Of maximal importance would be identifying behavioral signals which might indicate a latent readiness to adopt mobile payments. This depth of insight, obtained by sifting through billions of data points at lightning speed, would be inconceivable for human analysts to achieve without AI’s machine learning and information processing power.
dotData’s AI examined billions of multivariable data points across multiple datasets, including customer information, device specifications, transactional records, location data, caller history, and many other factors. From this, promising behavior patterns within the current mobile payment service adopters were identified and extrapolated to the larger pool of potential customers.
This intelligence allowed marketing executives and analysts to understand their customer base better and devise new promotional campaigns that hit home more effectively, resulting in a significant rise in conversions.
dotData’s system used machine learning capabilities to build behavioral models or avatars. The predictive profiles could then identify strong candidates amongst customers who had not yet used mobile payments. The client could even run the upselling check in real-time while a customer service or sales rep spoke to an existing customer.
How dotData Solved the Client’s Problem
dotData’s Feature Factory technology analyzed billions of data points across multiple categories such as customer data, call logs, transactions and recharges, account balances, device specifications, and even loyalty program usage. From this massive pool of data, the AI identified several unique patterns which demonstrated a correlation between certain behaviors and customer adoption of mobile payment systems.
The three main groups identified by their behavior patterns included several correlations the client would never otherwise have suspected. Here is a breakdown of the three groups:
- Customers who recharged their prepaid mobile calling service with credit cards were four times more likely to sign up for the client’s mobile payment service.
- Those who recharged their international calling credit regarding a specific country were ten times likelier to sign up for the mobile payment service.
- When a customer placed 50% or more of their calls to a specific country, the likelihood of conversion increased 500%
AI-Powered Customer Insights Lead to Conversion Boom
The behavioral patterns discovered by dotData, which earmarked certain customers for potential conversion to mobile payment services, allowed the client to develop much more focused sales and marketing campaigns. The hope was that such campaigns would increase their hitherto disappointing conversion rate.
And so it proved, with a conversion rate that increased by 1000%. In other words, the client achieved a tenfold increase in customers adding mobile payments to their accounts.
Furthermore, the client could now allocate marketing and sales resources much more effectively, resulting in fewer wasted calls and lowering the cost of sales. The call center received qualified leads rather than undifferentiated ones. Focusing on qualified leads meant that their campaigns were likelier to succeed and reduced negative responses from prospects.
By aiming tailored marketing campaigns at a pool of much more promising leads, the client increased conversions ten times more effectively than they had done using their previous scattergun approach. Once again, AI delivered meaningful insights to convey competitive advantage.
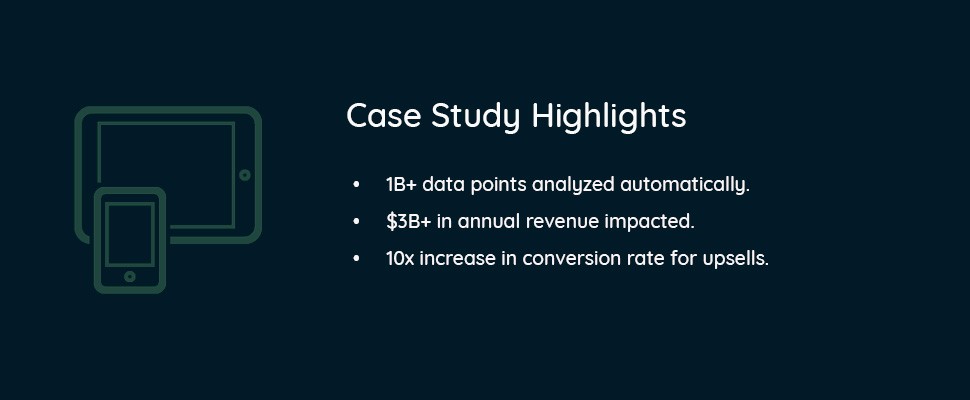
Share On