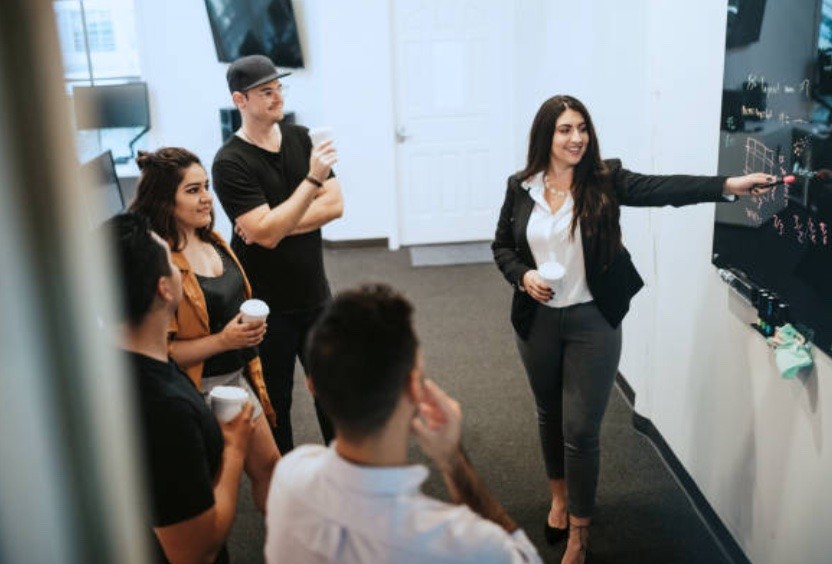
The Top 5 AI & Machine Learning Trends
Updated
The past couple of years have seen dramatic changes as the pandemic first drove the need for more online transactions and communications, then became the cause of worldwide shortages that have increased inflation and forced organizations around the globe to rethink their strategies. Machine Learning stands at the epicenter of these changes, but the long-term success of ML projects will be impacted by technology trends that continue to alter and shape the market for Machine Learning products and enabling technologies. This post is an updated version of an older post. Here are the latest trends we see as critical to ML practitioners:
Augmented Analytics Transforming Business Intelligence
Augmented Analytics will transform Business Intelligence – Augmented Analytics uses AI and ML technologies to assist with data preparation, insight generation, and explanation to expand how people explore and analyze data in analytics and BI platforms. AI is a critical enabling technology, and enterprises need an efficient way to scale their AI practices and implement AI in business. As organizations face increased pressure to optimize their workflows, more and more companies will ask BI teams to develop and manage AI/ML models. This drive to empower a new class of BI-based “AI developers” will be driven by two critical factors: First, enabling BI teams with AutoML platforms is more sustainable and scalable than hiring dedicated data scientists. Second, the life cycle from “requirement” to the working model will be accelerated because BI teams are closer to the business use cases than data scientists. More BI vendors will offer AI capabilities such as natural language processing, text analytics, and predictive dashboards, and AI + BI will be the new norm.
AI/ML Accessibility With No Code AI
No-code AI will make AI/ML accessible to a much broader swath of businesses and users. Organizations worldwide are investing in technologies that help them accelerate and democratize the data science process as the need for additional AI applications grows. Democratization implies empowering line-of-business, management, and operational teams with advanced analytical capabilities without requiring specialized data science skills using no-code AI. Many of these no-code platforms are workflow-driven, visual drag-and-drop tools that claim to help make AI easier for non-technical people. Although simple workflows are easy to build and conceptualize, the problem is that most AI/ML models require large, very complex, and sophisticated workflows that quickly become unwieldy and create new challenges of their own. The vast majority of the work that data scientists must perform is often associated with the tasks that precede the selection and optimization of ML models, such as feature engineering. Organizations must look for new, more sophisticated ML platforms that enable true no-code end-to-end automation. Automatically creating and evaluating thousands of features (AI-based feature engineering) and ML operationalization will be critical. No-Code AI platforms alone, however, will not be enough. Organizations embracing AI/ML for the first time will need to partner with vendors that can provide coaching, co-development, and support programs that guide new adopters through the early stages of AI/ML adoption.
See it in Action
See the power of Feature Engineering Automation with a personal demo.
Smart Manufacturing & Retail
AI/ML, Real-time Analytics, and IoT will enable Smart Manufacturing. The Covid-19 crisis disrupted supply chains, small and medium businesses were crushed, and shortages at grocery stores and online stores ran out of stock for essential items. As companies make recovery plans, manufacturers urgently need to be more resilient and transform operations using advanced technologies. Industry 4.0 initiatives will transition from PoCs to production. Diverse data will be analyzed automatically to find hidden patterns and uncover insights. Streaming analytics, aka stream processing, will enable manufacturers to make intelligent decisions with real-time applications such as predicting supply chain disruption or preventing unplanned downtime. Ubiquitous sensors and real-time quality monitoring will significantly reduce product recall as the manufacturing world embraces predictive and prescriptive analytics. The intersection of AI/ML, real-time analytics, and IoT will make manufacturing more efficient, resilient, and agile. As manufacturers and retailers exit from a high level of instability due to the pandemic, they will need continued agility for a rapidly changing economic climate. Models and automation that worked six months ago will be outdated quickly. Agility in model design, development, and deployment will be critical as data science teams are forced to accelerate experiments and iterate faster.
AI-Powered Automation
AI-powered Automation will trigger a new wave of innovation. The next digital transformation wave will use AI to optimize organizational efficiencies, generate deeper data-driven insights, and automate business decision-making. AI-enabled digital transformation will expand from “early adopters” such as financial services, insurance, and manufacturing to other industries. AI and ML will be embedded in multiple business functions across key business areas to drive efficiencies and create new products and services. The availability of automated ML platforms makes it possible for organizations to implement AI quickly and easily without investing in a data science team. As the Machine Learning platform and tools market continues to evolve, we expect considerable consolidation and specialization. Buyers will need to understand their business requirements and take stock of the sophistication of their ML development teams, their ML technology stack and where automation might make the most sense. In many cases, applying AI-powered automation to the early phases of AI/ML development – the data stage – will become a critical differentiator as organizations strive to “do more with less” and ask data science teams to iterate experiments faster and bring new and updated models to production quickly.
Fixing “the Data Problem”
As ML and AI become mission-critical across a wider range of industries, companies must find solutions to the “data” problem. Enterprise data is notoriously messy, complicated, and often massive in scale. Enterprises that want to make ML and AI an integral part of their strategy will need to find ways of automating as much of the data preparation workflow as possible, converting AI and ML development from an Artisanal approach to a product-line workflow that relies on intelligent automation not to replace talent but to improve efficiency, provide greater insights into data patterns and to allow the business to gain a higher return on investment from their AI/ML expenditures faster. Automating the process of converting data into feature pipelines for Machine Learning will be at the heart of this data transformation but will also provide significant benefits in associated areas like advanced analytics as automated feature discovery is leveraged to help businesses gain greater insights about their business in record time.
Responsible and Ethical AI Practices
Responsible AI, Explainability, and Model Interpretability will be critically important. The focus on bias in AI, regulatory, and privacy requirements will pave the way for more transparency in AI and ethical AI practices that build trust. As more organizations adopt AI into their business processes, there are concerns and risks about ML/AI models’ automated decisions. Interpretable features help organizations stay accountable for their data-driven decisions and meet compliance requirements. White-box models (WBMs) explain how they behave, produce predictions, and what variables influenced the model. With WBMs, AI is actionable, explainable, and accountable. Broader adoption of WBMs will empower enterprise model developers, model consumers, and business teams to execute complex AI projects with full confidence and certainty, building trust.