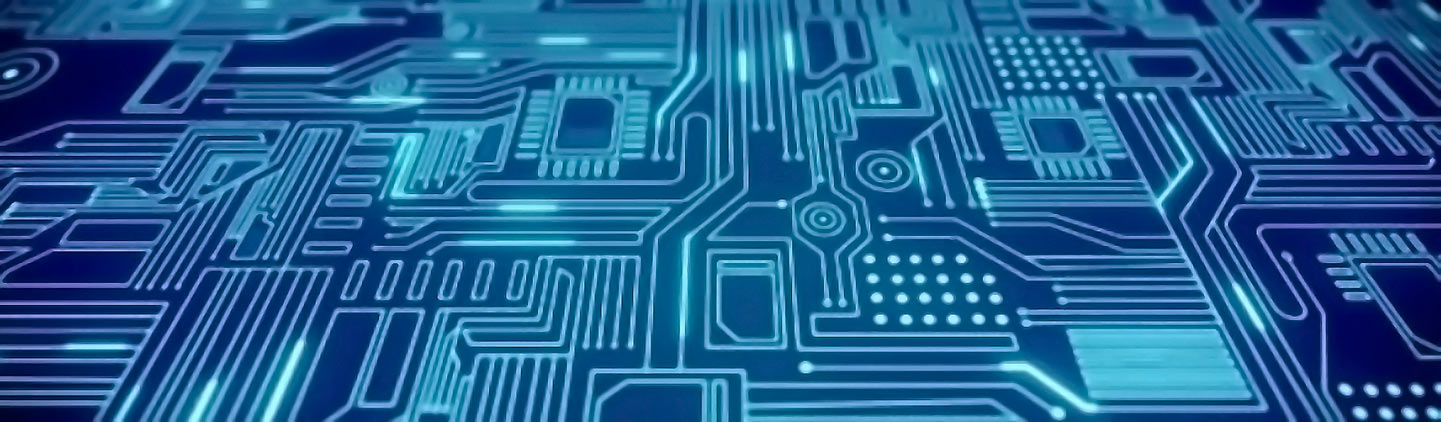
UX Defines Chasm Between Explainable vs Interpretable AI
The discussion about interpretability vs. explainability should start with why interpretability and explainability are important for various individuals,” our CEO Ryohei Fujimaki told @sEnterpriseAI’s @glawton: https://bit.ly/2ZAh5VT #ArtificialIntelligence #MachineLearning
AI explainability and AI interpretability are distinctions often used interchangeably but with very different applications. Explainable AI allows users to understand the models and can pass down the explanation to users. The distinction between interpretability and explainability is essential for various individuals. Interpretability applies to rules-based algorithms, and explainability applies to black-box deep learning algorithms. For users, developers, and C-suite members, providing explanations for AI/machine learning models boosts confidence in the models. Transparent models of machine learning can help make sense of the data, while explainable models can make sense of black-box models.
Black box models might look like a word cloud and weigh positive words more highly than negative words. Discussing how AI-UX fits into explainable vs. interpretable AI shifts focus from representation to explanation. When you want to use interpretable representations, use decision trees to represent an AI’s logic. Explainability is a process of making interpretations accessible to users, and self-explanation systems can present options and compare them in the context of an interactive dialog. There are two types of interpretability: explainability and interpretability. The goal of explainability is for an expert to be able to build and debug an AI application. Human users can’t understand everything, and interpretability helps them intervene when the system doesn’t make sense. The difference between explainable and black box models is essential when data scientists choose among different algorithms. If the performance is similar, it’s better to use the transparent approach for model inspection. Read more about this at EnterpriseAI.