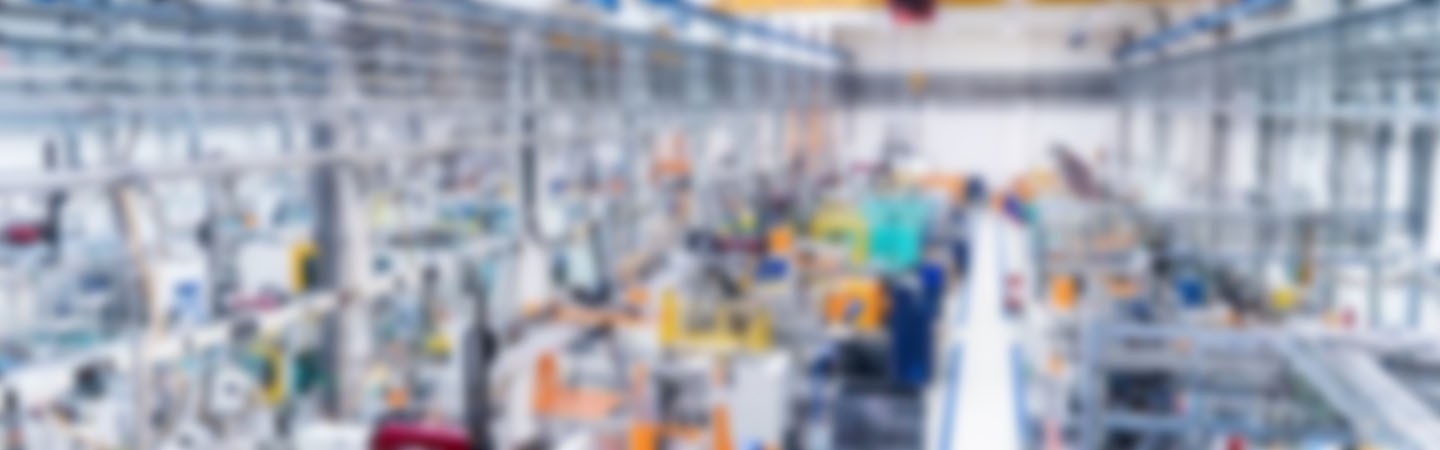
AI Automation To Enable Smart Manufacturing
Heavy industries such as Steelmaking are ramping up digital transformation initiatives to improve throughput, efficiency, safety, and reliability of their operations. Metals, mining, and machine tool building companies are embarking on multi-year journeys to digitize operations by adding connectivity, automation, and advanced analytics. According to McKinsey, most heavy-industry sectors are at the middle stages of digital maturity (Digital 2.0) relying on rule-based automation and distributed control systems. Some have made progress in digital maturity (Digital 3.0) and are using collaborative robots and advanced process control systems. However, a few digital pioneers are leveraging AI Automation and applying Machine Learning (ML) to operational data (Digital 4). These digital leaders offer powerful lessons that others can emulate and follow to successfully deploy ML at scale.
Drowning in Sensor Data
With the ubiquitous sensor network and pervasive connectivity in industrial manufacturing, data from production machines has continued to grow at an unprecedented scale. Manufacturing and process engineers need to review operational data across many sources such as ERP systems, MES, historians, computerized maintenance management systems, etc. Due to the sheer volume and streaming nature of data, manual inspection and analysis is not practical and unsustainable. Data from diverse systems come in multiple units, formats, and incompatible protocols. To get a better handle on data, companies have invested in building massive data warehouses, data marts, and data lakes, but that has brought new challenges about data governance and validation. Industrial operations teams need a scalable, secure, and high-performance environment for operational data management, review, learning, and analysis.
AI In Manufacturing
Implementing AI and ML techniques in manufacturing can be challenging for a variety of reasons:
- The standard AI workflow is complicated and laborious, with long lead times. A typical AI project involves data preparation, feature engineering, algorithm selection, model training, testing, and deployment and takes anywhere between 6-9 months.
- Getting quality data in a manufacturing environment is very difficult as each manufacturing site can have multiple ERP and manufacturing execution systems. With disparate systems, cleansing and aggregating data, building features, and generating models manually can become tedious, expensive, and labor-intensive. By the time you perform training and validation, AI/ML models have a high likelihood of being already outdated.
- The majority of AutoML platforms are designed for data science professionals and are not easy enough to use for manufacturing teams. These platforms have a steep learning curve and are cumbersome to use. Often the vendors don’t provide sufficient training or education in data science and how to implement AI for common use cases.
- With just-in-time production, many applications have low latency requirements that demand real-time processing. An ML system deployed under these tough operating conditions should have streaming analytical capabilities with the ability to process data under one second (often millisecond processing). A batch processing approach will not work.
- Manufacturing sites may not allow cloud-based analytics due to the sensitive nature of data. An on-premises, edge deployment for remote, resource-constrained production environments may be preferred. However, many vendors lack the ability to deploy models on the edge on small memory footprint such as edge servers or gateways.
Industrial IoT, manufacturing, in particular, requires real-time prediction capabilities for use cases such as quality monitoring and anomaly detection. A traditional modeling approach gets very complicated requiring significant investments in infrastructure.
AI Automation At Scale
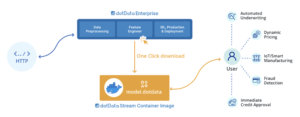
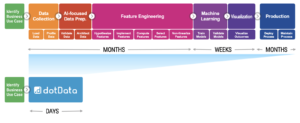
Any AI/ML solution for the manufacturing environment must be robust, flexible to address multiple use cases, and support real-time streaming capability. Moreover, it should offer the flexibility to deploy models in the cloud, on-premises, or at the edge depending on site requirements. The ideal solution should incorporate a platform designed and built for industrial manufacturing; a system that is usable by the operations team without requiring additional data science resources. A system that leverages operators domain expertise (Human-Centered AI) to augment performance.
Using a supervised ML technique, the system should find patterns in production data, identify deviation in quality, and empower SMEs to prevent recurrence. One of the biggest steel manufacturing companies in Japan, JFE Steel recently deployed dotData’s AutoML 2.0 full-cycle automation platform on their manufacturing plant. JFE Steel has a vision to deploy hundreds of various AI models to implement intelligent IoT Cyber-Physical Systems in their steel manufacturing plants and dotData Stream, our containerized ML model, is a key component to realize the vision at JFE Steel. Using AI automation, this system hides the pipeline complexities, automates feature engineering, and other onerous steps of the AI process and enables manufacturing customers to build predictive models in days instead of months.
To make AI transparent, it provides explanations and metrics about features with details into the modeling approach and ensemble of models selected. Explainable AI gives insight into predictions, allowing users to understand the correlations, and enables SMEs to understand which process variables affect the outcome for root cause analysis. Whether it is optimizing efficiency, preventing quality defects, or reducing asset downtime, industrial manufacturing presents multi-dimensional problems. And because the manufacturing environment generates so much data, AI is the perfect tool to solve manufacturing problems. A domain-agnostic, end-to-end AI platform offers analytical flexibility to address multiple use cases, and dramatically improves the life of operational experts as they don’t have to learn new tools for each new project. This is the preferred approach among digital pioneers as it saves time, allows repeatable ML processes, and delivers superior ROI.
AI automation allows SME to focus on day to day jobs, automate data pre-processing, and feature engineering and build models at the click of a button. Explainable AI and transparent features build trust and garner buy-in from domain experts. Containerized prediction model allows real-time prediction capability and accelerates AI deployment at the edge on the manufacturing floor. Empowering manufacturing and production SMEs to do more with less using AI automation is the way to achieve Digital 4.0 maturity level in the heavy industry at scale.
Share On