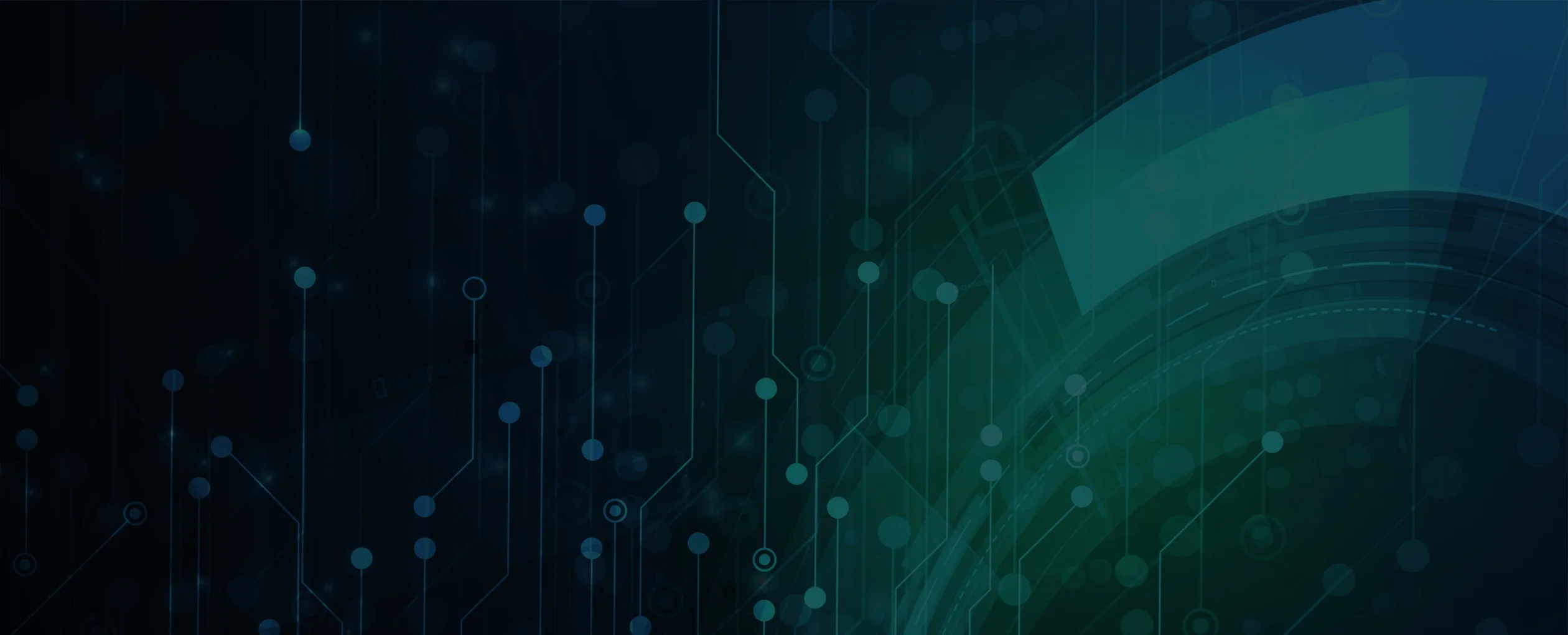
How AI is Reshaping the Lending Industry
- Industry Use Cases
A Guide for Chief Risk Officers Navigating the AI Revolution.
For Lenders, the way data is used for credit decision-making is undergoing a profound transformation driven by the convergence of Deep Analytics, Artificial Intelligence (AI), Machine Learning (ML), and Large Language Models (LLM). The combination of these technologies and the unprecedented explosion of available data has represented a significant opportunity in recent years.
While AI-powered technologies present substantial challenges for Chief Risk Officers (CROs), this shift also opens a future where the accuracy of risk prediction, understanding underlying drivers, and mitigating exposure to poor lending decisions move from a competitive advantage to becoming a core business requirement. Leaders at financial institutions face growing pressure to make accurate, fair, and expedient lending decisions while staying within the bounds of regulations and managing the headwinds of economic uncertainty.
This post explores how this AI-powered revolution reshapes credit risk prediction practices, offering actionable insights for leaders and practitioners. It’s a future where lending practices are not just improved, they’re transformed.
Financial institutions have huge volumes of rich and comprehensive data. While lenders have always accessed traditional sources like credit bureau data, alternative data like transactional information and utility payment data, just to name a few, can provide a rich, highly-granular mix of data that offers a wealth of signals but has been historically very difficult to analyze or include in analytics or risk models. This shift to a more diverse, richer data set brings opportunity and risk.
While a more comprehensive data set could increase accuracy and provide a deeper understanding of borrower behaviors and risk factors, it also requires more sophisticated and powerful tools to extract and evaluate patterns from that data, yielding meaningful signals that provide business value.
In addition, regulatory constraints like the Consumer Lending Act, the Credit Practice Rule, the Equal Credit Opportunity Act (to name a few), as well as data privacy regulations such as GDPR and the CCPA, complicate matters. Banks and lenders also often struggle with data silos that lead to fragmented information across departments and roles, creating roadblocks to creating more holistic views of risk and faster lending process. Research from Equifax found that using alternative data like rental payments, utility bills, and checking account information can score 8.4 million previously unscorable borrowers, so-called “thin-file” applicants, who may not have a poor credit history but also may not have a complete or compelling history either. These borrowers can benefit the most from using AI and ML in assessing credit risks, as these technologies can analyze a wide range of data to provide a more accurate risk assessment.
Legacy credit scoring models often relied on logistic regression or simple weighted scoring but were limited in capturing complex non-linear relationships that tend to exist in real-world data. AI and ML algorithms like gradient boosting, random forest, and neural networks can extract value from these intricate patterns, improving the accuracy of loan default and delinquency predictions and even helping with fraud detection. ML models should not be static but can be retrained and refined with new data, allowing them to adapt to changing market and economic conditions.
For example, an auto lender might use ML to discover that frequent address changes, the vehicle being financed, and patterns in utility or rent payments might be indicators of early payment defaults. This approach can create a proactive method of segmenting customers, adjusting underwriting criteria, and potentially reducing costs, losses, and processing time. While ML and AI can be powerful tools in the fight against credit defaults, the “black box” nature of some techniques can raise concerns around transparency and regulatory compliance. This is where Explainable AI (XAI) is critical. Technology that can provide XAI-based approaches to pattern discovery and model building can provide transparency into why a specific prediction was made, ensuring fairness, accountability, and the ability to explain decisions and models to regulators.
Large Language Models (LLMs) represent a leap forward in understanding and processing text. These models are trained on massive datasets and can perform sophisticated analysis of textual data, making them ideal in situations like sentiment analysis, going far beyond traditional keyword-based approaches and identifying the meaning trapped in conversations.
In the context of credit risk management, LLMs can unlock hidden signals trapped in loan applications, customer service chat logs, and other text-heavy content. An LLM process could effectively analyze thousands of customer service interactions to spot recurring complaints about a specific financial product’s terms and conditions, flagging hidden risk factors related to unclear communication or predatory lending practices.
In lending, LLMs can also automate the tedious, repetitive tasks of reviewing loan applications, ensuring compliance with lending regulations, while spotting potential red flags. By automating tedious and time-consuming reviews of text-heavy information, LLMs can increase the efficiency of risk analysis, accelerating the lending process.
Predictive models are the mainstay of forecasting and mitigating potential losses in the lending industry. Predictive models, however, often don’t provide a clear understanding of why those losses occur. On the other hand, deep analytics focuses on uncovering the causal relationship between data, which is the foundation of risk.
Leveraging AI deep analytics goes beyond simply identifying correlations and aims to understand how the interplay between signals can contribute to the historical reasons for borrower defaults. Techniques like business signal importance analysis (which reveals the relative contribution of discovered signals to the movement in a Key Performance Indicator) are powerful tools for this purpose.
For example, an automotive lender might use deep analytics to determine that, rather than a low credit score, a combination of high credit utilization and a recent uptick in late payments of utility bills is a stronger indicator of loan default within a narrow-but-essential customer segment. Discovering insights outside a narrow “predictable” pattern allows lenders to adjust underwriting and lending criteria for improved long-term performance. For example, the same lender could provide financial literacy programs to specific segments of clients or adjust loan terms to mitigate the identified risk. The key is to translate data-derived insights into actionable strategies that allow for the optimization of lending practices, operational efficiency and the improvement of overall portfolio performance.
Successfully traversing the evolving risk and technology landscape requires CROs and their respective teams to take proactive steps. Invest in a robust data infrastructure to handle the volume, variety, and complexity of modern data. In an environment where teams with deep expertise in AI, ML, and deep analytics are challenging to find and can be expensive to hire, identifying vendors that can provide highly differentiated technology solutions that can make the job of your existing analytics teams more straightforward and more efficient is also crucial. Prioritize explainable AI to maintain transparency and regulatory compliance. Establish strong data governance policies for continual high data quality and borrower privacy, and ensure the ethical use of AI in lending decisions. Finally, commit to an ongoing learning process as technologies in AI for lending continue to evolve.
The convergence of technologies like AI, ML, LLM, and Deep Analytics fundamentally reshapes how financial institutions and lenders predict and analyze risk in the lending industry. CROs who embrace AI in lending decisions and deepen their data-driven approach will position their businesses to thrive in a new era where increased access to data is the norm, regardless of market and economic conditions. By moving beyond traditional methods and leveraging the power of predictive analytics and advanced deep analysis, lenders can improve the accuracy of risk assessment and gain a deeper understanding of factors driving creditworthiness. The future of managing risks is here, driven by deep artificial intelligence.