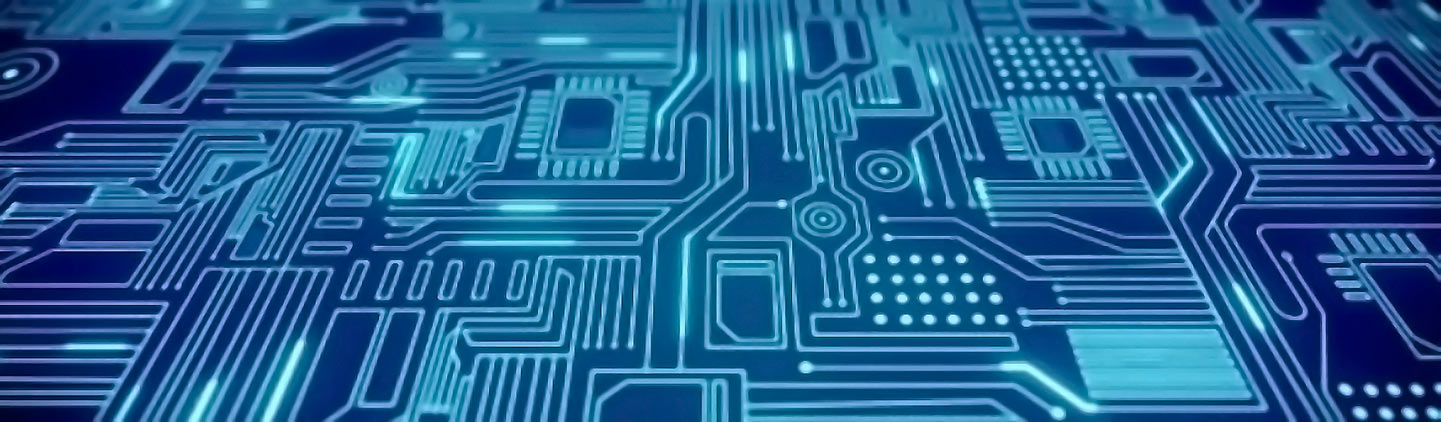
Can AI Enable Smart Factory and Realize Industry 4.0 Vision?
Accelerate Digital Journey for Manufacturers with AI Automation
Smart factory technologies that leverage the industrial internet of things (IIoT), edge computing, autonomous vision systems, and AI and Machine Learning can significantly improve cost, throughput, quality, safety and provide revenue growth. Despite the benefits, a recent Deloitte survey showed that only 5% of US manufacturers had fully converted at least one smart factory, while about 30% reported ongoing smart factory initiatives. AI is critical for Smart Factory enablement, and Implementing AI in industrial operations is challenging. Many solutions are designed for greenfield (new factories) projects and do not address complex data management, challenging legacy machinery integration, enterprise security requirements, real-time analytics, and the capability to handle thousands of models in the production environment. A fundamental problem is finding skilled people to develop and deploy AI. Global manufacturing leaders often rely on citizen data scientists – subject matter experts with domain knowledge in operations – and provide them with advanced analytical tools.
For Manufacturers to scale AI applications, the industry must address several challenges before AI-led digital transformation converts a factory into a Smart Factory:
- Think brownfield deployment first: Most manufacturing sites typically have a wide variety of machines, tools, and systems that use disparate and often competing technologies. For example, industrial robots from Kuka, ABB, and Fanuc dominate discrete manufacturing and require different skills to integrate, program, and troubleshoot. Industrial engineers often struggle with connecting legacy machines. They have to carefully think through connectivity options and find the right Industrial IoT gateway compatible with Ethernet, EtherCAT, MQTT, OPC UA, depending on the machinery used at the plant. The burden usually falls on system integrators to find sensors, protocol converters, and gateways they need to install. It should be relatively easy to connect to legacy applications – ERP, MES, and PLC/SCADA system. There is no easy way to collect data in the absence of universal connectivity at existing factories built 25-40 years ago, hence no AI and ML applications.
- Address data management issues – Operational data from the production floor lives across multiple databases in multiple formats, not suitable for analytics. In the manufacturing setting, access to reliable data makes data management issues more critical given the harsh operating conditions and noisy environment. How do you ensure you have high-quality data for AI/ML projects? As an example, a predictive maintenance solution will need data from a computerized maintenance management system, process historians, and operational data logs or databases. The manufacturing site may also be remote, bringing additional complexities in terms of data storage. The IT team may not want to send data to the cloud, requiring on-premises solutions. The traditional approach companies take to solve any data issues requires months of effort. The time and the initial efforts associated with this approach often cause projects to fail relatively quickly. To avoid these problems, AI/ML platforms that provide AI-focused Data Preparation, Feature Engineering automation, ML automation, and automated production are the future of data science for data-driven manufacturers. End-to-end data science automation involves automating the entire data science process and addresses data management issues, and renders faster delivery of insights from months to days.
- Real-Time Analytics is necessary – Many manufacturing applications are sensitive to latencies, such as predictive maintenance or predictive quality, and require an ultra-fast response. For these applications, the system cannot wait for the round trip journey to the cloud to perform data processing and get actionable insights. The decision has to be made in real-time, acted upon in a few milliseconds. Real-time data processing allows manufacturers to take action immediately and prevent undesirable consequences. For example, by using predictive analytics for quality, manufacturers can identify defective components and perform rework or replace the faulty part containing a product recall. Real-time decision making and ultra-fast response time require edge-based computing architecture. The ability to deploy predictive models on the edge devices such as machines, local gateway, or server is critical to enable smart manufacturing applications.
- Explainable AI is a must – The AI technology stack is extraordinarily complex and challenging. Without a data science background, people struggle to understand how predictive modeling works and do not trust AI technology’s abstract algorithms. Transparency implies providing information about the AI pipeline – the input data used in the process, algorithms selected, and how the model made predictions. One approach to increase trust is to provide details about the AI workflow. That includes providing a detailed process to transform the raw data into machine learning inputs (a.k.a. feature engineering) and how the ML model produces predictions by combining hundreds of or even more features. Lack of transparency is where Explainable AI can help. By giving insight into how the prediction models work and the reasoning behind predictions, manufacturing organizations can increase transparency and remove the bias against AI.
See it in Action
See the power of Feature Engineering Automation with a personal demo.
Digital Transformation leaders should look for collaborative AI tools – products, solutions that leverage and augment the SME’s domain knowledge. Smart factories need predictive analytics solutions designed for the manufacturing team – intuitive, easy to learn and use, and one that offers simple integration with existing infrastructure. Providing shop floor engineers with advanced analytical tools will empower them to drive data-driven operational improvements and build the foundation to create value from AI at scale. A collaborative AI platform that automates data preparation offers automated feature engineering, and promotes interpretability with explainable AI is the need of the hour in smart manufacturing.
By leveraging a collaborative AI platform and AI automation, you can connect factory assets, processes, and people to realize the smart factory vision where manufacturing is agile, responsive, and self-healing.
To learn more about AI/ML workflow, top challenges, and how to overcome them, here is a white paper on the practical difficulties of enterprise AI: Practical Challenges of Enterprise AI