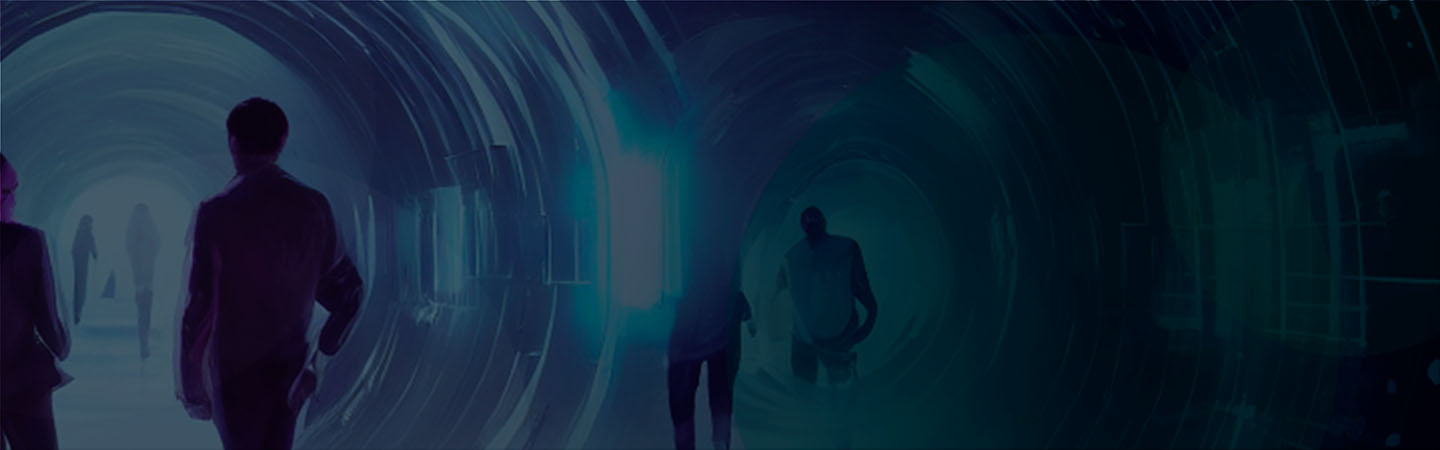
Predictive vs. Prescriptive Analytics: What is the Difference?
- Thought Leadership
Predictive and prescriptive analytics are two forms of advanced analytics. Predictive analytics helps us predict future outcomes, whereas prescriptive analytics takes those predictions to the next level by identifying the likely outcomes of these predictions and suggesting ways to alter them. Predictive analytics is understanding what will happen, and prescriptive analytics is understanding how to make it happen, prevent it, or change it.
Predictive analytics is a form of advanced analytics that can help a business predict future outcomes. Predictive analytics can predict customer behavior, future trends, or the likely outcome of current activities. The predictions are derived by analyzing historical data and using statistical methods to predict the outcomes we can expect in the future.
Churn prediction detects which customers a business is likely to lose, for example, through the cancelation of a subscription to services. Churn prediction can provide insight into possible future earnings changes and give an organization a high degree of focus to try and prevent the churn from happening in the first place. Churn prediction is one of the most popular use cases for predictive analytics.
In the hospitality or entertainment industry, predictive analytics can predict staffing levels or product demand needed on a given day. Understanding staffing trends can help manage and allocate resources more efficiently and answer questions such as the expected demand for services across all locations in an upcoming period or the expected number of guests in a hotel on any given weekend or holiday period.
Machine learning engineers can use medical data to diagnose and predict patient illness. An increase in consumer wearable technologies, such as smartwatches that can provide a wealth of data about the patients, has resulted in additional data that can lead to improved results through better and more accurate predictions. Predicting sickness can reduce hospitalizations, ER visits, invasive procedures, and many more.
Predictive analytics provides a great deal of value when implemented properly. Through the ability to predict likely business outcomes, leaders can better forecast resource allocation, understand trends that are relevant to the business, and react to changing market conditions with a higher degree of confidence.
Analyzing historical patterns and trends in conjunction with the predictions can help lead to concrete business actions that can drive meaningful business value. This process is called prescriptive analytics and is the final stage of successfully making data-driven decisions with a predictive model.
Banks can use prescriptive analytics to suggest what to do to ensure customers are retained. By acting promptly, a bank can provide attractive offerings to upsell clients before they potentially churn. Prescriptive models can help provide insights into why some customers churn and can provide recommendations on the mix of services and products that are most likely to reduce the likelihood of churn.
In the healthcare community, prescriptive analytics can gain insights into the causes of patient illnesses and can be used to make recommendations for the best courses of action to alter the progress of a disease, interventions that are likely to provide the greatest degree of success, and medications that might provide relief. Prescriptive analytics in healthcare is typically only used as a recommendation system.
One of the critical metrics in sales is lead scoring. Lead scoring takes into account various data, such as email communication, content engagement, and page views, to calculate a lead score, which predicts the likelihood of the lead becoming our customer. Based on the insights from the previous customers and the prediction results, marketers can apply recommended steps to increase the lead score and increase the probability of leads becoming customers.
The business value of prescriptive analytics stems from its ability to predict outcomes and provide insights into how to impact those outcomes. When a business knows that current processes and procedures are not successful, it allows them to proactively improve them. Prescriptive analytics helps understand what should be done differently and how those changes are likely to impact future performance.
Prescriptive analytics is challenging and has to be approached carefully. Different variations of a business decision may result in different outcomes. The variability of prescriptive analytics means that predictions may lead in a direction we didn’t expect – or desire. While good prescriptive analytics tries to encompass all of this, it is challenging.
The most crucial difference between predictive and prescriptive analytics is the analyzed data. Predictive analytics uses historical data to uncover hidden patterns, which are then used to make good predictions. Prescriptive analytics uses historical data, the results of the projections, and the hidden patterns discovered during the predictive analysis to take business action and recommend further steps.
The second key difference is that predictive analytics finds correlations in the data, while prescriptive analytics involves finding causal relationships. There can be 100 weekly correlated reasons in a complex equation that can accurately predict customer churn, but which of those 100 reasons should the business take action to see a substantial business value? Finding causation makes prescriptive analytics much harder since the business hypotheses created from the predictions may or may not yield acceptable business value.
The third key difference is that prescriptive analytics cannot exist without predictive analytics. Business value can be derived by deploying predictions since those still give us insights based on which we can make decisions. Prescriptive analysis is a deeper dive into the projections and what drives them; therefore, it cannot exist independently.
The combination of predictive and prescriptive analytics is the holy grail of data-driven decisions. Predictive analytics can exist without prescriptive analytics, but the most value can be obtained from your predictions when you understand their factors and can influence them to drive real business change! Learn how to use dotData to drive predictive analytics with end-to-end data science automation.