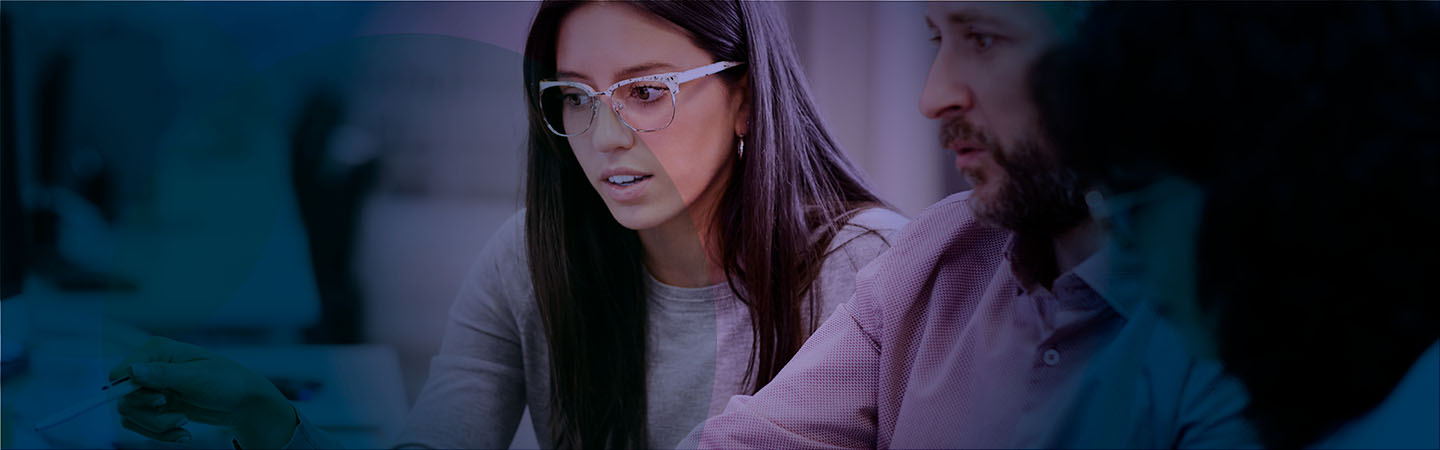
Component Supplier Lowers Bad Debt $15M With Machine Learning Credit Risk Assessment
- Customer Stories
This US distributor of electrical components needed to predict potential credit defaults during the pandemic, but with 10,000+ clients, doing it manually was – at best – challenging.
dotData’s Managed Predictive Analytics program gave their BI team the training and software to build ML models using a 100% no-code approach.
With dotData, the BI team created a model in 90 days. Centralized credit risk assessment saved the company over $15M per year.
The client is one of the US’s largest electrical component distributors with 150 locations and over 2000 employees. Annually, the company makes more than $1 billion in revenue from its over 10,000 B2B customers.
Many customers are small to medium businesses (SMBs) with variable and unpredictable revenue patterns, meaning they don’t always pay invoices within the statutory terms. This creates a heavy financial burden on accounts receivable (AR) departments. This aggregated debt risk is highly time-consuming to service using manual processes and debt managers.
Accounts receivable is one of the most vital departments in any B2B retail business. Ensuring that a steady revenue stream offsets expenditure is crucial to the ongoing profitability of a company. But SMBs are becoming ever more erratic in their invoice payment behavior, exacerbated by the parlous state of the US economy following the COVID-19 pandemic.
According to the PYMNTS/American Express joint report, 15% of B2B debt is in default. Unpaid invoices created a significant liability for the client, amounting to around $1 million of unrecovered debt per month. With over 150 locations generating invoices, managing bad debt regionally became challenging with the volume of overdue payments.
In the first year of the pandemic (Mar 2020 to Feb 2021), over 200,000 businesses were forced to close because of reduced trade and revenue, despite the Biden administration’s SMB support measures. Even setting aside COVID-19 consequences, the US has seen over 600,000 businesses fold in recent years.
A survey by Atradius revealed a startling 72% increase in year-on-year invoice defaults since the pandemic began. Suppliers polled by Atradius reported that 43% of invoices were overdue, demonstrating a widespread liquidity problem amongst customers.
Ideally, the regional credit managers would be able to predict defaulting accounts and generate early warnings about customers who were about to go into unacceptable levels of debt. However, given the scale of the problem, and the labor-intensive nature of invoice chasing, this had not been possible when pursued as a purely manual process.
The client had a two-fold problem on their hands: Increasing defaults on overdue accounts and indefinite non-payments from some near-bankrupt businesses.
While dealing with non-paying SMBs, the client also experienced an upsurge in trade from smaller resellers of electrical tools benefiting from government support for the construction business. It was impossible to assess bad debt risk without access to historical payment records. It became essential for the client to find new and advanced methods for estimating risk.
The sheer volume of customer and credit control data which would have to be analyzed regularly, also presented a challenge. Regional credit managers could only focus on the larger accounts, using legacy credit scoring methods and occasionally reporting “at-risk” customers back to the head office.
The ad hoc process functioned mainly on the regional credit managers’ experience and “gut instincts.” This method could not scale to work for the thousands of smaller vendors and resellers who formed the bulk of the company’s debtors. There weren’t enough managers to cover the customer base, and scaling the workforce was not economically viable.
Inevitably, thousands of small, defaulting accounts were left largely unmanaged due to this dilemma.
dotData realized that they could harness the power of dotData’s pattern discovery and analysis technology to spot the early warning signs of soon-to-be-defaulting accounts. The client would be able to draw upon a host of data sources to spot signals of credit risk before they were self-evident. Credit risk signals would indicate that a particular customer stood a strong chance of defaulting on payment.
Traditional credit scoring methods focused on a handful of static data sources, providing a snapshot in time without tracking account behavior to find underlying patterns behind the data.
By contrast, a credit modeling system trained with dotData’s technology could analyze millions of data points across many sources, including customer data, historical invoice payments, store data, expenditure patterns, etc. dotData allowed the client to build a highly effective predictive model of future behavior.
An ML model built with dotData could draw insights from vast pools of data that lay beyond the capabilities of human resourcefulness. Having access to these early warning signs, clients could then take steps to intervene before a likely problem became a crisis.
Planned interventions included requiring part payment upfront or sending out invoices as early as possible to allow for eventual payment delays, lowering the likelihood of accounts receiving 90-day default notices.
Using dotData’s predictive AI modeling, the company reduced its annual bad debt by US$15 million. The reduced bad debt equated to a 1% increase in annual revenue for the company while improving the balance sheet considerably. Compared with human analysis, AI modeling proved more effective than experienced debt managers in identifying risks and countering bad debt issues.
In addition, the system was able to identify and incorporate over 400 recurring patterns (features) of insights that accurately predicted debt risk and were incorporated into future automated risk procedures.
dotData’s automated feature engineering (AutoFE) technology accessed many data sources, including billing information, customer details and behavior, and individual store and regional data analyses. Combining these disparate sources helped highlight a particular issue some credit managers had spotted – critical patterns of repeated underpayment.
Integrated within automated payments and invoicing systems, dotData’s derived risk rating meant that credit managers could concentrate their efforts elsewhere. For example, larger clients’ high-risk transactions could become the focus of human inquiry, while dotData’s AI handled 80% of the invoicing and reimbursement issues.
The AI system’s ability to scrutinize invoicing and payment behavior on an ongoing basis meant that the company could identify problems or high-risk profiles as soon as relevant evidence appeared. Repetitive manual analysis of historical data gave way to automated early warning indicators.
The client was able to incorporate AI and machine learning into its tech stack to ensure the adoption of best practices on an ongoing basis. The company would see continuous revenue savings as dotData’s AI-generated models would continue to spot trends and adapt to changing economic circumstances.
The solution wasn’t a quick fix or an emergency measure but a valuable risk reduction measure, enabling our client to protect corporate revenues across all regions.
dotData solves the biggest challenge for organizations of all sizes: Turning raw business data into valuable insights ready for use in Machine Learning (ML) and Artificial Intelligence (AI) models. dotData provides unique products and solutions tailored to organizations just getting started with predictive analytics and more mature businesses with established data science and data engineering processes.
Our core technology automatically converts data from data warehouses and data lakes into feature tables by exploring the relationships between varied data tables with hundreds of columns and millions of rows.
Forrester recognized dotData as a leader in ML and AI in 2019, and CRN named dotData to its Big Data 100 list four years running. dotData was named a CB Insights Top 100 AI Startups for 2020 and was recognized as the “best machine learning platform” for 2019 by the AI breakthrough awards.
Fortune 50 companies around the Globe rely on dotData to help them accelerate their ML, AI, and Advanced Analytics projects. For more information, visit www.dotdata.com, and join the conversation on Twitter and LinkedIn.